Best Ai Software for Engineering
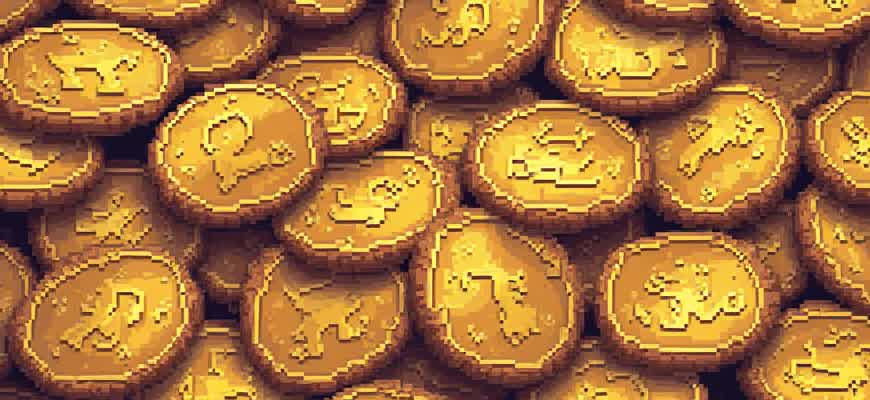
In the rapidly evolving field of engineering, the integration of artificial intelligence (AI) has become essential for improving efficiency, optimizing designs, and enhancing precision. With the rise of AI-driven software, engineers can leverage advanced algorithms to solve complex problems that were once time-consuming and error-prone. The following list highlights some of the most advanced AI tools that are transforming the engineering landscape.
Key Benefits of AI in Engineering:
- Enhanced design optimization
- Predictive maintenance and failure analysis
- Automated data analysis and simulation
- Increased accuracy in modeling and simulations
Popular AI Tools for Engineers:
- TensorFlow: Widely used for machine learning and deep learning applications, TensorFlow supports complex calculations in neural networks, making it ideal for predictive maintenance and anomaly detection.
- AutoCAD with AI Integration: AutoCAD’s AI features help streamline design processes, optimize structure layouts, and reduce human error in blueprint creation.
- MATLAB with AI Toolboxes: MATLAB’s AI toolboxes offer powerful simulation and modeling capabilities, enabling engineers to process large datasets and implement machine learning techniques seamlessly.
"AI in engineering isn't just about automation; it's about revolutionizing how engineers approach challenges, enabling faster, more efficient problem-solving and design optimization."
Comparison Table of AI Tools:
Tool | Primary Features | Best for |
---|---|---|
TensorFlow | Machine learning, deep learning, predictive analytics | Large-scale data analysis, anomaly detection |
AutoCAD | AI-powered design automation, structure optimization | Engineering design, architecture |
MATLAB | Simulation, data processing, AI toolboxes | Data analysis, modeling, machine learning integration |
Best AI Solutions for Engineering: A Comprehensive Guide
Artificial Intelligence (AI) has become a game-changer in engineering, enhancing design processes, simulation capabilities, and optimizing overall productivity. The integration of AI into engineering software tools has allowed for more efficient problem-solving, predictive maintenance, and data-driven decisions. In this guide, we will explore some of the top AI software solutions that are revolutionizing the engineering industry, especially with an eye towards the growing impact of blockchain and cryptocurrency technologies.
Blockchain technology, a key player in the crypto world, has also found its applications in engineering. AI software that integrates blockchain can secure data, ensure traceability, and streamline supply chain processes. Let’s explore some of the best AI tools for engineers working at the intersection of these transformative fields.
Top AI Tools for Engineering in the Crypto Space
- Autodesk Revit: Known for its capabilities in building information modeling (BIM), Autodesk Revit leverages AI to improve designs and workflows. In the context of cryptocurrency-related engineering, it can be used to model secure blockchain infrastructures.
- SolidWorks: This CAD software incorporates AI to optimize designs and simulations. It helps engineers streamline the creation of blockchain hardware systems by simulating various crypto mining hardware components.
- IBM Watson IoT for Engineering: Watson is ideal for building smart, secure systems that integrate both AI and blockchain. This tool is valuable for engineers developing decentralized applications (dApps) in the crypto space.
Key Features of AI in Engineering for Blockchain and Cryptocurrency Applications
- Automated Design Optimization: AI can automatically adjust designs to meet specific parameters, saving time and reducing human error in complex engineering tasks.
- Predictive Maintenance: With AI’s predictive capabilities, engineers can forecast when blockchain hardware or systems may fail, preventing costly downtime.
- Enhanced Security: AI can help identify vulnerabilities in blockchain networks and implement proactive security measures to protect against cyber threats.
"The convergence of AI and blockchain in engineering is not just about enhancing processes; it's about creating entirely new possibilities for secure, decentralized systems."
Comparison of Top AI Software Tools for Engineering
Software | AI Features | Best For |
---|---|---|
Autodesk Revit | AI-assisted modeling, real-time collaboration | Building information modeling (BIM) for blockchain systems |
SolidWorks | Design optimization, AI-based simulations | Crypto mining hardware design and prototyping |
IBM Watson IoT | AI-driven analytics, predictive maintenance | Smart system design with blockchain integration |
How Artificial Intelligence is Transforming Engineering Design Processes
Artificial Intelligence (AI) is rapidly reshaping engineering design, offering unprecedented levels of efficiency and innovation. From automating repetitive tasks to optimizing complex simulations, AI-driven tools are enhancing every stage of the design process. As engineers work to develop next-generation products, AI helps them focus on high-value tasks by reducing time spent on routine or error-prone activities.
By integrating AI, companies are able to streamline their workflows, generate more accurate models, and make smarter decisions. AI not only supports design but also enhances collaboration across multidisciplinary teams, ensuring that design decisions are data-driven and precise. The following outlines how AI software is revolutionizing engineering design.
Key Ways AI Transforms Engineering Design
- Automation of Repetitive Tasks: AI tools can perform routine tasks like data entry, error-checking, and preliminary design iterations, freeing engineers to focus on innovation.
- Predictive Design Optimization: AI algorithms can analyze vast amounts of data to predict optimal design parameters, reducing the need for trial-and-error approaches.
- Advanced Simulation and Testing: AI-based software can simulate various design scenarios in real-time, allowing engineers to explore performance outcomes under different conditions before physical testing.
- Collaborative Platforms: AI enhances communication across global teams by providing centralized platforms that track design changes and offer instant feedback based on real-time data.
Impact on Design Efficiency
"By incorporating AI, design cycles are reduced significantly, enabling faster prototyping and product iteration, while ensuring precision and innovation."
AI Feature | Impact on Design Process |
---|---|
Automated Prototyping | Reduces lead times and increases design iterations with fewer manual interventions. |
Data-Driven Insights | Provides engineers with real-time, data-backed design decisions, improving accuracy and functionality. |
Integrated Collaboration Tools | Fosters cross-functional communication and ensures all team members are aligned in design goals. |
Key Features to Look for in AI Tools for Engineering Simulations
In the world of engineering simulations, AI tools are becoming increasingly vital to improve efficiency, reduce errors, and enhance design precision. When selecting AI software for simulations, it is essential to focus on specific features that enhance computational capabilities, optimize workflows, and support the modeling of complex systems. Below are the main aspects to consider for engineers seeking to integrate AI into their simulation processes.
Whether it's fluid dynamics, structural analysis, or optimization tasks, AI-driven solutions should possess particular characteristics that ensure accuracy, scalability, and adaptability to diverse engineering problems. These features are critical to not only executing tasks with higher precision but also offering time-saving capabilities across various stages of the design and simulation cycle.
Key Features to Focus On:
- Advanced Machine Learning Integration: Look for AI tools that incorporate machine learning algorithms to predict and optimize complex patterns. These algorithms should be able to adapt to new input data and refine simulation accuracy over time.
- Real-Time Data Processing: The ability to handle real-time data and feedback during simulations is critical for iterative engineering processes. Tools should allow engineers to modify parameters instantly and see immediate results.
- Multi-Physics Simulation Capabilities: AI tools that support multi-disciplinary simulations (e.g., fluid-structure interaction or electromagnetic analysis) offer a more comprehensive view of the system behavior.
- Automated Optimization: A feature that enables AI to autonomously test and optimize various design parameters, ensuring the best outcomes with minimal human input.
Additional Considerations:
- Scalability: The tool should be able to handle large-scale simulations without compromising speed or performance.
- Interoperability: Ensure the AI tool is compatible with other engineering software and platforms commonly used within your organization.
- Ease of Use: Even with advanced capabilities, the user interface should remain intuitive to avoid a steep learning curve.
"Choosing an AI tool with the right blend of speed, adaptability, and multi-disciplinary support can significantly streamline your engineering simulation workflows and improve results."
Comparing AI Tools for Engineering Simulations:
Feature | Tool A | Tool B | Tool C |
---|---|---|---|
Real-Time Data Processing | Yes | No | Yes |
Multi-Physics Support | Yes | Yes | No |
Machine Learning Integration | No | Yes | Yes |
Optimization Features | Yes | Yes | No |
Enhancing Engineering Accuracy through AI Integration in Cryptocurrency Models
AI technologies are revolutionizing the way engineering models are developed and refined, especially in cryptocurrency networks where precision is paramount. The application of machine learning algorithms enables the optimization of blockchain protocols, ensuring that transaction verification, network stability, and data integrity are achieved with higher precision. These models utilize vast datasets and intricate calculations to forecast potential outcomes and enhance system performance, making them indispensable for decentralized finance (DeFi) and smart contract development.
Integrating AI in engineering models, particularly in blockchain-based ecosystems, allows for rapid detection of anomalies and precise adjustments to system variables. This is crucial in environments where speed and reliability are essential, such as in cryptocurrency mining algorithms or cryptographic security protocols. By using AI, engineers can reduce errors, increase scalability, and optimize energy efficiency, improving both the security and functionality of cryptocurrency networks.
Key Benefits of AI-Driven Precision in Engineering Models
- Data Analysis: AI enables advanced analytics that can process massive amounts of blockchain data, offering insights that enhance transaction validation and miner operations.
- Scalability: AI algorithms optimize resource distribution, allowing blockchain platforms to handle growing transaction volumes with minimal latency.
- Energy Efficiency: Machine learning models can predict and minimize the energy consumption of cryptographic calculations, benefiting the overall sustainability of cryptocurrency operations.
AI in Engineering Models for Cryptographic Security
"AI's ability to predict potential vulnerabilities in cryptographic systems makes it an invaluable tool in securing blockchain networks. Machine learning models enhance the encryption process, providing stronger resistance against emerging attack vectors."
Examples of AI Integration in Cryptocurrency Engineering
- Smart Contract Optimization: AI algorithms are used to predict and prevent errors in smart contract execution, ensuring security and accuracy in decentralized applications (dApps).
- Mining Algorithm Improvement: Machine learning techniques enhance the efficiency of mining operations by predicting the most profitable blocks to mine, based on current network conditions.
- Transaction Verification: AI-based models help to speed up the validation of transactions while ensuring the integrity of data in decentralized ledgers.
Impact of AI on Blockchain Performance
Feature | Traditional Methods | AI-Enhanced Methods |
---|---|---|
Transaction Speed | Moderate | High |
Energy Consumption | High | Optimized |
Scalability | Limited | Enhanced |
How AI Improves Predictive Maintenance in Engineering Projects
Predictive maintenance has become a cornerstone in engineering due to the growing need for efficient asset management and reduced operational downtime. By utilizing AI algorithms, engineering teams can analyze large amounts of sensor data to detect patterns and anomalies in real-time, leading to more accurate predictions on when equipment is likely to fail. This capability significantly improves the decision-making process, allowing for timely interventions and reducing maintenance costs.
AI-driven solutions for predictive maintenance incorporate machine learning, advanced data analytics, and IoT sensors to enhance the reliability of machinery and infrastructure. By continuously monitoring equipment and processing historical performance data, these tools can provide actionable insights and forecasts. This process not only minimizes unplanned outages but also extends the life of critical components by ensuring they are maintained before costly breakdowns occur.
Key Advantages of AI in Predictive Maintenance
- Real-time Monitoring: AI continuously tracks operational data, enabling instant identification of potential failures.
- Cost Reduction: Predictive maintenance helps in minimizing emergency repairs and the associated costs.
- Efficiency Improvement: Engineers can plan maintenance based on the actual condition of equipment, ensuring minimal disruptions to operations.
- Optimized Resource Allocation: Resources are utilized more efficiently by focusing maintenance efforts on equipment that requires attention.
How AI Processes Data for Maintenance Forecasts
- Data Collection: Sensors installed on equipment capture continuous performance metrics.
- Data Processing: AI models process this data to detect patterns or deviations from normal operation.
- Failure Prediction: The system identifies when a component is likely to fail based on historical data and trends.
- Maintenance Alert: Alerts are triggered to notify engineers of the need for preventative action.
"AI’s ability to predict maintenance needs is transforming the way engineering projects are managed, reducing unexpected failures and extending the lifespan of key assets."
Comparing AI-Driven Predictive Maintenance Tools
Tool | Technology | Key Feature |
---|---|---|
IBM Maximo | Machine Learning & IoT | Real-time asset health monitoring |
Uptake | AI & Predictive Analytics | Condition-based maintenance alerts |
Predikto | Big Data & Machine Learning | Asset performance forecasting |
Choosing AI Software for Automating Engineering Calculations
When it comes to automating engineering calculations, the use of artificial intelligence has become a game changer. Engineers can leverage AI-powered tools to simplify complex calculations, reduce human error, and improve overall efficiency. These tools can help streamline repetitive tasks, such as structural analysis or fluid dynamics simulations, freeing up engineers to focus on more strategic problem-solving.
However, selecting the right AI software for engineering calculations involves understanding specific technical requirements and workflows. Different software tools offer varying features, ranging from simulation capabilities to integration with existing CAD systems. Making an informed choice ensures that your team gets the most out of the automation process, resulting in more accurate outputs and faster project completion times.
Key Features to Look for in AI Engineering Software
- Automation of Repetitive Calculations: Ensure that the software can handle repetitive calculations across different engineering fields, such as thermal, structural, and electrical analyses.
- Advanced Simulation Capabilities: Look for AI tools that provide high-performance simulations, whether for fluid dynamics, stress testing, or other complex scenarios.
- Ease of Integration: The software should seamlessly integrate with existing CAD and simulation software, minimizing the learning curve for your team.
- Scalability: As your project grows, the software should be capable of handling increasing amounts of data without compromising performance.
- Data Security and Compliance: Ensure the software meets industry-specific compliance standards and offers strong data protection mechanisms, especially when dealing with sensitive calculations.
Popular AI Solutions for Engineering
- MATLAB with AI Toolbox: Known for its versatility in engineering calculations, MATLAB offers advanced AI and machine learning toolboxes to automate and optimize engineering designs.
- ANSYS AI: ANSYS integrates machine learning and AI into its simulation platforms, allowing for advanced predictive maintenance and optimization of engineering processes.
- Simulink AI: A part of MATLAB, Simulink uses AI for automating systems and simulation-based design, making it an ideal tool for control engineering tasks.
AI in engineering not only accelerates calculations but also improves accuracy by identifying patterns and optimizing processes that would be challenging for traditional methods.
Comparison of AI Software for Engineering Calculations
Software | Primary Strength | Best For |
---|---|---|
MATLAB | Extensive libraries and toolboxes | Complex algorithmic calculations and data analysis |
ANSYS | Advanced simulations and predictive analysis | Stress testing, fluid dynamics, and material science |
Simulink | Model-based design and control systems | Engineering system modeling and automation |
Impact of AI on Collaboration Across Engineering Teams
The integration of AI technologies is transforming the way engineering teams interact and collaborate. Automation and data-driven insights are helping teams streamline their workflows, improving overall productivity and reducing errors. AI systems are now capable of providing real-time feedback and suggestions, which allows teams to make faster and more informed decisions during the design and testing phases of projects.
AI also plays a significant role in breaking down traditional barriers between different engineering disciplines. By enabling seamless communication and data sharing, AI fosters interdisciplinary collaboration. Engineers from various domains can now work together in a more integrated way, leveraging AI-powered tools to collaborate on complex designs and solve problems more effectively.
AI Tools Enhancing Teamwork
- Real-Time Collaboration Platforms: AI tools allow engineers to share and update designs in real time, enabling teams to work simultaneously on the same project.
- Intelligent Data Analytics: AI-powered analytics tools process vast amounts of data to provide actionable insights, helping teams make better-informed decisions and optimize their designs.
- Automated Problem-Solving: AI can automatically identify potential issues in designs or processes, reducing the need for manual checks and speeding up the problem-solving process.
Key Benefits of AI for Engineering Collaboration
Benefit | Description |
---|---|
Improved Efficiency | AI accelerates design iterations and reduces bottlenecks by automating repetitive tasks. |
Enhanced Communication | AI tools provide easy access to shared data, improving communication among team members from different disciplines. |
Faster Decision-Making | AI offers real-time insights that allow teams to make data-driven decisions quickly, minimizing delays in the design process. |
"AI is not just a tool for individual engineers but an enabler for collaborative, interdisciplinary innovation."
AI Tools for Faster Time-to-Market in Engineering Solutions
In the engineering industry, minimizing time-to-market is a critical factor in staying competitive. AI-powered tools are increasingly being adopted to streamline the design, simulation, and testing processes, accelerating product development. By automating repetitive tasks and optimizing workflows, AI allows engineers to focus on innovative solutions while reducing human error and inefficiencies.
When it comes to engineering applications in cryptocurrency, the pressure to develop and deploy products swiftly is even greater. Blockchain-based projects require continuous adaptation and fine-tuning of algorithms, ensuring security, scalability, and performance. AI solutions are particularly valuable in this field, offering tools for predictive analysis, intelligent automation, and real-time optimization.
How AI Reduces Time-to-Market
AI enhances the engineering process in multiple ways:
- Automated Design and Prototyping: Machine learning algorithms can generate design models quickly and predict their viability, saving time compared to traditional trial-and-error methods.
- Predictive Maintenance: AI-driven monitoring systems help prevent downtime by predicting failures and suggesting fixes before problems arise.
- Real-time Data Analysis: AI tools analyze massive amounts of data in real-time, enabling quicker decision-making and faster problem resolution during product development.
AI Tools in Blockchain and Cryptocurrency Engineering
The use of AI in blockchain and cryptocurrency development offers significant benefits, especially in reducing time-to-market for innovative solutions. Below are some AI-driven tools that are transforming the industry:
Tool | Purpose | Benefits |
---|---|---|
AI Smart Contracts | Automates the contract execution process | Reduces errors, increases efficiency |
Blockchain Analytics | Real-time transaction analysis | Identifies issues quickly, reduces risk |
AI-Powered Cryptocurrency Trading Bots | Automates trading strategies | Speeds up market response, increases profits |
AI tools help reduce the time-to-market in engineering solutions by optimizing complex processes, automating manual tasks, and offering predictive insights, ensuring faster delivery of reliable, scalable products in the cryptocurrency space.