Ai Powered Trading Algorithms
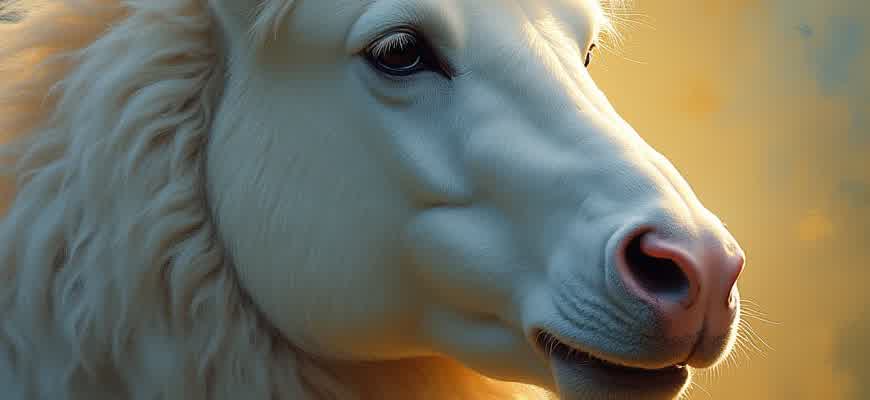
The integration of artificial intelligence into cryptocurrency trading is transforming the way investors and traders approach market analysis and decision-making. By utilizing machine learning models and data-driven insights, AI-powered algorithms can process vast amounts of market data and execute trades with remarkable precision, adapting to the ever-changing conditions of the crypto market.
These advanced algorithms are designed to recognize patterns, predict market movements, and automate trading strategies based on historical data. Below is an overview of how these algorithms function:
- Market Data Analysis: AI algorithms continuously analyze real-time market data to identify trends, price fluctuations, and sentiment shifts.
- Pattern Recognition: Machine learning models are trained to detect patterns in price behavior that may suggest future market movements.
- Trade Execution: Based on the analysis, the algorithm automatically executes buy or sell orders, optimizing for factors like timing and pricing.
Key advantages of AI-driven trading systems include:
- Increased Efficiency: AI can process data and make trading decisions faster than human traders, allowing for more efficient market participation.
- Reduced Emotional Bias: Unlike human traders, AI algorithms do not suffer from psychological factors like fear or greed, ensuring objective decision-making.
- 24/7 Operation: AI-powered systems can operate around the clock, taking advantage of global market fluctuations without interruption.
"AI-powered trading algorithms not only enhance the accuracy of market predictions but also eliminate the need for manual intervention, allowing for a truly autonomous trading experience."
Benefit | Description |
---|---|
Speed | AI can execute trades in milliseconds, much faster than a human could react. |
Accuracy | Machine learning models improve their accuracy over time by learning from past trades. |
Risk Management | AI algorithms can manage risk through dynamic stop-loss and take-profit strategies. |
AI-Powered Algorithms: Enhancing Cryptocurrency Trading Strategies
Artificial intelligence has revolutionized the way cryptocurrency traders approach the market. By analyzing vast amounts of data in real-time, AI-powered trading algorithms can provide insights and predictions that human traders might miss. These systems use advanced machine learning techniques to identify patterns, predict price movements, and automate trades. With cryptocurrency markets being highly volatile, the integration of AI into trading strategies has proven to be a game-changer for investors looking for a competitive edge.
AI-driven algorithms are not just reactive; they can be programmed to learn from past data, adapt to market shifts, and optimize strategies continuously. By reducing human bias and emotion, AI algorithms ensure that trades are executed with precision and consistency. This has made them indispensable tools for both novice and experienced traders looking to maximize their returns in the highly unpredictable world of cryptocurrency.
How AI Improves Cryptocurrency Trading
AI-powered trading systems bring numerous advantages to investors. Below are the key benefits of using AI for trading in the cryptocurrency market:
- Data Analysis: AI algorithms can process large datasets and market indicators in real-time to identify trends that humans might overlook.
- Automated Trading: AI can execute trades automatically based on pre-set criteria, eliminating the need for constant monitoring of the market.
- Risk Management: AI can help identify potential risks and adjust trading strategies accordingly to mitigate losses.
- Continuous Learning: Machine learning enables algorithms to evolve and adapt, improving their accuracy over time.
Important Considerations When Using AI for Crypto Trading
While AI-powered trading systems offer substantial benefits, there are also several factors to consider before relying on them for your cryptocurrency investments:
- Market Complexity: Cryptocurrencies are volatile, and AI can sometimes misinterpret the impact of external factors like news or regulation changes.
- Over-Optimization: Algorithms that perform well in historical data may not always perform well in future conditions, especially in dynamic markets.
- Security: Using AI algorithms requires ensuring that your trading systems are secure from cyber threats and market manipulation.
"AI trading algorithms are a powerful tool, but they are not foolproof. Their success heavily depends on the quality of the data and the strategy behind them."
AI-Powered Trading Strategies
The table below outlines several common AI-powered strategies used by cryptocurrency traders:
Strategy | Description |
---|---|
Arbitrage Trading | AI identifies price discrepancies across different exchanges to profit from the difference. |
Sentiment Analysis | AI analyzes social media and news to gauge market sentiment and predict price movements. |
Trend Following | AI tracks market trends and executes trades based on the direction of the market. |
How AI Algorithms Analyze Cryptocurrency Market Trends in Real-Time
AI-powered trading systems are designed to leverage vast amounts of data to predict price movements in the cryptocurrency market. These algorithms utilize machine learning techniques to analyze historical price data, trading volumes, and other relevant metrics in real-time. By continuously updating their models based on incoming data, AI systems can quickly identify trends and adjust strategies accordingly, offering traders an edge in a highly volatile market.
Real-time analysis of market conditions is crucial for profitable cryptocurrency trading. AI algorithms use a variety of methods, such as natural language processing (NLP) to analyze news sentiment and deep learning to recognize complex patterns in price movements. By processing data at a much faster rate than human traders, AI systems can identify trading opportunities that might otherwise go unnoticed.
Key Methods Used by AI in Real-Time Market Analysis
- Data Mining: AI algorithms mine vast datasets to extract patterns, correlations, and insights from both structured and unstructured data sources.
- Sentiment Analysis: By analyzing news articles, social media, and forums, AI algorithms can gauge market sentiment, helping to predict market shifts.
- Pattern Recognition: Machine learning models are trained to recognize complex price patterns, enabling predictive analysis of future price movements.
- Reinforcement Learning: Some AI systems use reinforcement learning techniques to continuously optimize their trading strategies based on real-time feedback.
Example: AI Decision-Making in Action
Time | Price | Sentiment | Action Taken |
---|---|---|---|
10:00 AM | $45,000 | Positive news sentiment | Buy Signal |
10:15 AM | $45,500 | Market stability | Hold |
10:30 AM | $44,800 | Negative sentiment | Sell Signal |
AI-driven trading algorithms can adapt to rapidly changing market conditions and adjust their strategies based on new data, often outperforming human traders in terms of speed and accuracy.
Optimizing Risk Control with AI in Crypto Trading Systems
As the cryptocurrency market is highly volatile and prone to rapid fluctuations, integrating artificial intelligence into risk management strategies has become crucial. AI-powered algorithms can analyze vast amounts of data and identify patterns in real time, making it possible to predict market movements and optimize trading decisions with a level of precision that human traders cannot achieve alone.
By leveraging AI for risk management, trading systems can dynamically adjust their strategies based on ongoing market conditions, continuously minimizing the likelihood of significant losses. Machine learning models can be used to detect emerging risks and alter trade parameters, ensuring that investments stay within predefined risk limits while maximizing potential returns.
Key Techniques for AI-Based Risk Optimization
- Real-Time Risk Assessment: AI systems continuously monitor market data and calculate risk scores, adjusting exposure levels accordingly.
- Portfolio Diversification: By analyzing correlations between various digital assets, AI algorithms can automatically diversify portfolios to minimize the impact of any single asset’s volatility.
- Stop-Loss & Take-Profit Automation: Machine learning models predict optimal stop-loss and take-profit levels, adjusting them dynamically to protect against unexpected price swings.
Types of AI Algorithms for Risk Management
- Reinforcement Learning: This algorithm learns from market interactions and refines risk management strategies through trial and error, continually improving over time.
- Neural Networks: These models can process non-linear relationships in price movements, identifying hidden risks and making predictions about potential market downturns.
- Decision Trees: AI can use decision trees to evaluate different risk scenarios and choose the most appropriate actions based on historical data and current market conditions.
Example Risk Management Table
Risk Factor | AI Optimization Approach | Outcome |
---|---|---|
Market Volatility | Dynamic adjustment of exposure using real-time data | Reduced drawdown during volatile periods |
Liquidity Risk | AI-driven portfolio diversification across assets | Improved asset liquidity and reduced slippage |
Unexpected Price Movement | Real-time stop-loss and take-profit orders | Minimized losses in sharp price declines |
"AI offers a transformative approach to risk management, allowing crypto traders to stay ahead of market trends and avoid significant losses during periods of high volatility."
Integrating AI Algorithms into Your Cryptocurrency Trading Platform
AI-driven trading algorithms can significantly enhance the efficiency of cryptocurrency trading, providing real-time data analysis and decision-making capabilities that are far beyond the scope of traditional methods. Integrating AI with your existing trading platform allows you to leverage automated strategies, predict market trends, and optimize trading decisions. However, implementing AI algorithms requires a well-thought-out approach to ensure seamless integration and maximize performance.
To successfully integrate AI algorithms, it's crucial to evaluate your platform's compatibility with AI models, ensure sufficient data processing capacity, and establish robust communication channels between the AI system and your existing infrastructure. The following steps can help guide the integration process.
Steps to Integrate AI with Your Trading Platform
- Assess Platform Compatibility: Evaluate your platform’s architecture and determine whether it supports the computational needs of AI models, such as data processing power and real-time capabilities.
- Data Preprocessing and Pipeline Setup: Establish a data pipeline to ensure smooth data flow between your trading platform and the AI algorithms, ensuring clean and structured input data.
- API Integration: Use APIs to link AI-driven decision-making tools with your trading platform, enabling real-time trading actions based on AI predictions.
- Testing and Optimization: Before going live, run simulations to test how the AI model interacts with your platform. Continuously optimize the system for performance improvements.
AI Algorithm Components in Crypto Trading
AI Component | Role in Trading |
---|---|
Sentiment Analysis | Analyzes social media, news, and market sentiment to predict price movements. |
Predictive Models | Generates price forecasts using historical data and statistical techniques. |
Reinforcement Learning | Adapts trading strategies in real-time by learning from market behavior. |
Integrating AI into cryptocurrency trading can revolutionize how decisions are made, transforming the traditional rules-based approach into dynamic, data-driven strategies.
The Role of Machine Learning in Enhancing Algorithmic Trading Accuracy
In the world of cryptocurrency trading, machine learning (ML) has revolutionized the way algorithms operate, enhancing their predictive accuracy and response time. As markets become more volatile and unpredictable, the integration of advanced ML techniques into trading algorithms allows for faster and more informed decision-making, improving the overall performance of trading strategies. These algorithms are capable of analyzing vast amounts of historical and real-time data, identifying patterns, and making predictions with greater precision than traditional methods.
Machine learning models, especially those using supervised and unsupervised learning, have proven to be instrumental in refining algorithmic strategies. By continuously learning from data and optimizing their predictions, these models adapt to market shifts, ensuring that the trading algorithm remains competitive in the face of ever-changing conditions. ML enhances the ability to detect subtle correlations between various market signals, which can otherwise be overlooked in manual analysis.
Key Benefits of Machine Learning in Algorithmic Trading
- Real-Time Decision Making: ML models process real-time market data, allowing algorithms to make quick, data-driven decisions without human intervention.
- Pattern Recognition: Through deep learning, ML can identify non-obvious market trends and predict price movements based on historical data.
- Improved Accuracy: The continual training of models ensures better accuracy in trade predictions, reducing the risk of costly errors.
How Machine Learning Improves Trading Algorithm Performance
- Data Processing: ML algorithms can sift through massive amounts of unstructured data, extracting meaningful insights from news, social media, and other sources.
- Adaptive Learning: As the market changes, machine learning models update themselves to reflect new conditions, which allows for ongoing strategy optimization.
- Risk Management: By assessing past performance and market behavior, machine learning helps mitigate risks by adjusting trading strategies dynamically.
"Machine learning enables trading algorithms to outperform traditional methods by learning from large datasets and adapting to changes in the market, ultimately enhancing profitability and reducing risk."
Example of an ML-based Trading Strategy
Algorithm Type | Description | Benefit |
---|---|---|
Reinforcement Learning | Uses reward-based systems to optimize trading strategies by continuously improving decisions based on past actions. | Enables adaptive decision-making in volatile markets. |
Neural Networks | Simulates human brain processes to detect intricate market patterns and price predictions. | Improves pattern recognition and forecasting accuracy. |
Advantages of Using AI-Driven Algorithms in Cryptocurrency Trading
Automating cryptocurrency trading with AI-based algorithms offers several compelling advantages for investors and traders. These algorithms can execute trades with high precision and speed, allowing for better risk management and the potential for higher returns. The ability to continuously analyze market data and adjust strategies in real-time is a significant asset in a highly volatile market like cryptocurrencies.
AI-powered trading systems can adapt to changing market conditions, making them highly effective in unpredictable environments. They remove human emotion from the decision-making process, which often leads to better, more consistent outcomes. Let’s explore the key benefits of automating crypto trades using advanced AI algorithms:
Key Benefits
- 24/7 Market Monitoring: AI algorithms can monitor cryptocurrency markets around the clock, ensuring no trading opportunity is missed, even when human traders are offline.
- Faster Execution: These systems can react to price movements and execute trades within milliseconds, much faster than human traders can manually react.
- Data-Driven Decision Making: AI algorithms analyze massive datasets from multiple sources, enabling them to make more informed decisions compared to human traders relying on limited information.
Impact on Performance
- Consistent Results: Automation eliminates emotional trading decisions, leading to more consistent and predictable outcomes over time.
- Optimized Risk Management: AI algorithms can adjust stop-losses and take-profit levels dynamically based on market conditions, helping to minimize potential losses.
"The ability of AI to analyze vast amounts of market data quickly allows traders to capitalize on short-term price movements that might otherwise be missed."
Comparison of Traditional vs AI-Based Trading Systems
Feature | Traditional Trading | AI-Powered Trading |
---|---|---|
Speed of Execution | Manual, slower response | Instantaneous, millisecond accuracy |
Emotion Management | Emotional decisions based on fear or greed | Emotion-free, logic-based decisions |
Market Monitoring | Limited by trader's availability | Continuous 24/7 market analysis |
How AI-Driven Trading Systems Adjust to Market Instability
In the world of cryptocurrency, market fluctuations are a common phenomenon, and AI-driven trading systems are designed to respond dynamically to these changes. By leveraging advanced algorithms, these systems are able to identify patterns in real-time data, allowing them to make informed trading decisions even in volatile conditions. The key challenge for AI models is to continuously adapt to rapidly shifting market sentiments, which are heavily influenced by factors such as news, social media trends, and macroeconomic events.
AI-powered trading strategies utilize multiple data points, from historical prices to sentiment analysis, to predict potential market movements. Machine learning models are particularly effective in this regard, as they can continuously retrain on new data, improving their accuracy over time. However, in highly volatile markets like cryptocurrency, the models must remain flexible to quickly adjust to sudden price swings and unexpected news events.
Key Strategies for Adapting to Volatile Markets
- Real-Time Data Processing: AI systems rely on fast data input from multiple sources, including market orders, social media, and news outlets. By processing this information in real-time, the system can respond quickly to emerging trends.
- Predictive Modeling: Through deep learning and reinforcement learning, AI models can forecast price movements based on historical data, adjusting their predictions as market conditions evolve.
- Sentiment Analysis: AI systems analyze social media and news sentiment to gauge market psychology and adjust trading strategies accordingly.
"AI algorithms are designed to continuously evolve, using real-time feedback to improve their decision-making ability in the face of market unpredictability."
Adaptive Learning Mechanisms
Machine learning-based trading algorithms adjust by using continuous feedback loops. These loops allow the model to fine-tune its approach based on recent performance, improving accuracy in the face of new data. For example, a reinforcement learning model may revise its strategy after every trade, learning from both wins and losses.
Example of an AI-Driven Trading Algorithm Performance
Market Condition | AI Response | Outcome |
---|---|---|
High volatility (Price Spike) | Quick adaptation with smaller position sizes and increased risk management | Minimized losses and capitalized on trend shifts |
Low liquidity | Holds trades longer to avoid sudden liquidations | Stable returns despite low activity |
Understanding the Data Inputs that Drive AI Trading Decisions in Cryptocurrency
AI-powered trading systems leverage a vast array of data to make highly informed decisions within the volatile world of cryptocurrency markets. The accuracy and efficiency of these algorithms heavily depend on the quality and range of the inputs they receive. These inputs can be grouped into several categories, each playing a unique role in enhancing the model's predictive power and overall performance.
The primary data sources that guide the trading algorithms in cryptocurrency include historical market data, real-time market signals, social media sentiment, and blockchain-specific metrics. These inputs provide AI models with a comprehensive view of the market's behavior, which in turn helps in making calculated predictions. Understanding these data points is critical for developing effective trading strategies.
Key Data Inputs for Cryptocurrency AI Trading
- Price and Volume Data: Historical price and trading volume data are essential for identifying market trends and price patterns.
- Market Sentiment: AI systems analyze real-time news, social media, and forums to gauge public sentiment towards a particular cryptocurrency.
- On-Chain Metrics: Metrics such as transaction volume, wallet activity, and hash rate are crucial for assessing the health and stability of a blockchain network.
- Macro Economic Indicators: Global financial trends, interest rates, and inflation data are also relevant as they can influence investor behavior.
Important: AI models rely on a diverse set of data inputs. Without accurate and timely data, the algorithm may miss critical market movements, leading to suboptimal decisions.
Example of Data Inputs in AI Trading Models
Data Type | Description |
---|---|
Price Data | Historical price trends, open/close prices, and price volatility. |
Volume Data | Trading volume that indicates market liquidity and investor activity. |
Social Media Sentiment | Analysis of public sentiment from platforms like Twitter and Reddit. |
Blockchain Data | Metrics such as transaction count, network activity, and block size. |
The more refined the data inputs, the more accurate the AI-driven trading decisions will be. By continually feeding relevant, high-quality data into the system, traders can maximize the effectiveness of AI algorithms in the fast-paced world of cryptocurrency markets.