Etf Using Ai to Pick Stocks
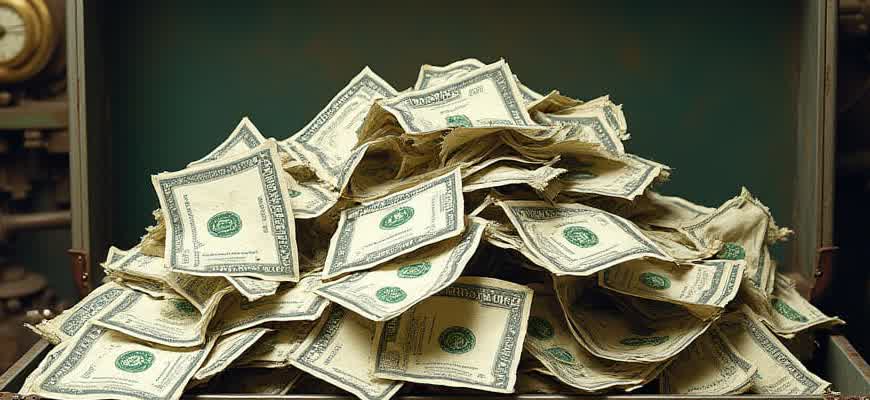
In recent years, exchange-traded funds (ETFs) have evolved beyond traditional passive investment strategies, with artificial intelligence (AI) playing a significant role in optimizing stock selection. Rather than relying on human intuition or broad market indices, AI-driven ETFs employ advanced algorithms to identify and invest in stocks with the highest potential for growth. By processing vast amounts of market data in real-time, these funds aim to outperform conventional investment methods.
AI systems in ETFs leverage a variety of factors when determining which stocks to buy, including:
- Historical price trends
- Sentiment analysis from news and social media
- Financial performance metrics
- Market volatility and liquidity indicators
Some of the key benefits of AI-managed ETFs include:
- Improved decision-making through data-driven insights
- Reduced human bias in stock selection
- Faster reaction times to market changes
Important: AI-driven ETFs are continuously learning from new data, which means their stock selection strategies evolve over time to adapt to market conditions.
Aspect | AI-Driven ETF | Traditional ETF |
---|---|---|
Stock Selection | Data analysis, predictive modeling | Market indices, human discretion |
Speed of Adjustments | Real-time updates | Periodic rebalancing |
Adaptability | Continuous learning | Fixed strategy |
Leveraging AI for Cryptocurrency ETF Selection: A Guide to Smart Investment
In the rapidly evolving cryptocurrency market, the use of artificial intelligence (AI) for stock selection is gaining significant traction. Cryptocurrency ETFs (Exchange-Traded Funds) that utilize AI are revolutionizing how investors approach digital asset investment strategies. By analyzing vast amounts of market data, AI models can identify trends, assess risks, and predict future price movements with remarkable precision, all of which are crucial in such a volatile space. This approach allows investors to diversify their portfolios with the added benefit of automation and predictive analysis, which is difficult for human traders to replicate manually.
AI-driven cryptocurrency ETFs combine cutting-edge technologies like machine learning and natural language processing to filter out noise and identify high-potential investment opportunities. These funds analyze various data points including transaction volume, on-chain activity, social media sentiment, and macroeconomic factors that influence crypto prices. The goal is to create a portfolio of digital assets that balances risk and potential reward in a way that traditional methods often overlook. Here’s a closer look at how AI is transforming crypto ETF strategies.
How AI Enhances Cryptocurrency ETF Selection
AI-driven ETFs use complex algorithms to select the most promising cryptocurrencies based on data inputs such as:
- Market Trends: AI evaluates historical price movements and forecasts future trends to determine entry and exit points.
- Blockchain Metrics: On-chain data, such as transaction volume and wallet activity, helps identify strong-performing cryptocurrencies.
- Social Sentiment: Sentiment analysis tools scan social media platforms to gauge market sentiment and anticipate price movements.
- Regulatory News: AI can track global regulatory developments that may affect the value and legitimacy of cryptocurrencies.
By processing these inputs, AI-driven ETFs aim to offer more precise predictions than human traders can achieve on their own. This can lead to better risk management and optimized returns.
Important Note: While AI provides powerful tools for predicting market movements, it is important to understand that cryptocurrency markets are inherently volatile, and no model can guarantee profits in the long term.
Steps to Investing in an AI-Powered Crypto ETF
Investing in AI-powered cryptocurrency ETFs involves several key steps:
- Research: Begin by understanding how the ETF uses AI. Review the underlying algorithms, the data sources, and the strategies employed by the fund.
- Diversification: Check the portfolio distribution across various digital assets. A well-diversified ETF mitigates risks in case of drastic market shifts.
- Risk Management: Ensure the ETF uses robust risk management techniques, especially given the unpredictable nature of crypto markets.
- Monitor Performance: Keep track of how the ETF performs over time. Evaluate the AI model's ability to adapt to market changes.
Performance Metrics of AI Crypto ETFs
AI-driven cryptocurrency ETFs often measure success using the following metrics:
Metric | Description |
---|---|
Volatility | The fluctuation in price of the cryptocurrency assets in the ETF portfolio. |
Return on Investment (ROI) | The percentage increase in value over a defined period. |
Sharpe Ratio | Measures the risk-adjusted return of the ETF, helping assess the quality of the investment strategy. |
How Artificial Intelligence Optimizes ETF Construction by Analyzing Stock Data
Artificial Intelligence (AI) has rapidly transformed the way investors approach building Exchange-Traded Funds (ETFs). By utilizing advanced algorithms, AI systems are capable of processing vast amounts of stock market data to identify patterns, predict trends, and select assets for ETF portfolios. These AI models analyze historical price movements, market sentiment, and financial reports, enabling them to make more informed investment decisions compared to traditional methods.
The integration of AI into ETF strategies provides investors with smarter, data-driven portfolios that are dynamically adjusted based on market conditions. Unlike conventional ETFs, which are often passively managed and based on predetermined indexes, AI-powered ETFs are actively optimized to align with evolving market signals. This adaptability significantly improves the risk-return profile of the fund and enhances overall performance.
Key Features of AI in ETF Construction
- Data-Driven Decision Making: AI systems process both structured and unstructured data from various sources, such as financial statements, news articles, and social media, to gain a comprehensive understanding of stock behavior.
- Predictive Analytics: AI leverages machine learning algorithms to predict future stock price movements based on past data, identifying which stocks are likely to perform well in the future.
- Real-Time Adjustments: AI can continuously monitor market fluctuations and adjust the ETF portfolio in real-time, ensuring the fund is always aligned with the latest trends and opportunities.
How AI Improves ETF Performance
"AI-driven ETF strategies reduce human biases, enhance diversification, and increase adaptability, leading to superior performance during volatile market conditions."
Incorporating AI into ETF management can lead to more efficient portfolio construction. By using machine learning models, AI can assess various factors, including volatility, correlation, and market liquidity, to build more robust and diversified funds. Additionally, AI can help detect early signals of market shifts, enabling faster reactions to changes in market sentiment.
Example of AI in ETF Construction
ETF Name | AI Model Used | Key Focus |
---|---|---|
AI-Driven Growth ETF | Deep Learning | Targeting high-growth stocks by predicting future performance |
Sentiment AI ETF | Natural Language Processing (NLP) | Analyzing market sentiment from social media and news |
The Role of Machine Learning in Stock Selection for ETFs
Machine learning (ML) is becoming a critical tool in the asset management world, especially in the selection of stocks for exchange-traded funds (ETFs). By utilizing large datasets and sophisticated algorithms, ML models can identify trends and patterns that human analysts may overlook. These systems are capable of processing vast amounts of data in real-time, providing fund managers with actionable insights to improve investment strategies. The application of ML techniques is revolutionizing how ETFs are structured, leading to more efficient and data-driven approaches to stock selection.
One of the most impactful aspects of ML in ETF management is its ability to enhance predictive accuracy. Unlike traditional methods, which rely heavily on historical performance and basic fundamentals, machine learning can continuously adapt and improve its predictions as new data is fed into the system. This dynamic approach is particularly valuable in volatile markets, including cryptocurrency, where price movements can be highly unpredictable and influenced by a range of complex factors.
Key Techniques Used in ML for Stock Selection
- Supervised Learning: This technique involves training the model on historical data to predict future stock prices based on input features such as earnings reports, macroeconomic indicators, and technical signals.
- Unsupervised Learning: This method helps identify hidden patterns or groups within data, which can be used to detect new market trends or emerging sectors for potential investment.
- Reinforcement Learning: This approach allows algorithms to optimize trading strategies by learning from past actions and their consequences in the market.
Applications in Cryptocurrency
Machine learning is also gaining traction in the cryptocurrency space, where traditional stock selection methods are often ineffective. Due to the volatile and relatively nascent nature of digital assets, ML is uniquely positioned to assess non-linear correlations and predict future price movements with greater accuracy.
"Incorporating machine learning into cryptocurrency ETF management can help identify undervalued digital assets, predict price fluctuations, and manage risk more effectively."
Technique | Application in Crypto ETF |
---|---|
Supervised Learning | Predicts the future value of a cryptocurrency based on historical data and news sentiment analysis. |
Unsupervised Learning | Clusters similar cryptocurrencies to identify emerging trends or market shifts. |
Reinforcement Learning | Optimizes asset allocation based on past performance and market conditions. |
Challenges and Opportunities
- Data Quality: The effectiveness of ML models depends on the quality of data. In the cryptocurrency market, data can be noisy, inconsistent, and prone to manipulation.
- Model Overfitting: There's a risk that a machine learning model could be too closely fitted to historical data, making it less effective in real-world scenarios.
- Regulatory Uncertainty: The evolving regulatory landscape for cryptocurrencies may impact the effectiveness of machine learning algorithms in predicting market trends.
Key Factors AI Considers When Selecting Stocks for an ETF
In the ever-evolving world of cryptocurrency investments, AI-driven algorithms have gained prominence for their ability to analyze vast amounts of data and make informed decisions. When it comes to selecting stocks for a cryptocurrency-focused ETF, AI relies on multiple factors to ensure optimal portfolio construction. These factors are not only based on market trends but also on deep analysis of blockchain technologies and the underlying value of digital assets.
The AI system typically incorporates several key indicators to determine which cryptocurrencies or blockchain-related stocks will provide the best risk-adjusted returns for the ETF. It uses historical data, technical signals, and even sentiment analysis to evaluate the potential of each asset. In doing so, the AI ensures that the ETF is comprised of assets that align with both the short-term and long-term market trends.
Factors Considered by AI in Cryptocurrency ETF Selection
- Market Liquidity: AI evaluates the liquidity of an asset, ensuring it can be easily bought or sold without significant price disruption. High liquidity is vital for smooth ETF operation and for minimizing trading costs.
- Volatility Analysis: Cryptocurrencies are known for their high volatility. AI gauges how volatile an asset is and adjusts ETF composition to mitigate unnecessary risks. The goal is to balance high volatility assets with more stable ones.
- Technology Assessment: AI considers the underlying blockchain technology, evaluating its scalability, security, and adoption rate. Projects with strong development communities and technological advancements are prioritized.
- Sentiment Analysis: The AI system scans social media platforms, news outlets, and forums to assess public sentiment towards various crypto assets. This helps predict market movements based on public perception.
Key Performance Metrics in AI Stock Selection
- Return on Investment (ROI): AI looks for assets with consistent and promising ROI projections, considering both historical performance and future growth potential.
- Risk to Reward Ratio: The AI balances risk factors with potential rewards, ensuring that the portfolio’s risk level aligns with investor preferences.
- Market Sentiment Indicators: AI tools analyze social media, news trends, and blockchain community feedback to gauge market sentiment and price movement predictions.
"The accuracy of AI predictions in cryptocurrency ETF selection heavily relies on continuous data input, machine learning models, and up-to-date market insights. Regular adjustments are crucial for maintaining an effective portfolio."
Comparison Table of Cryptocurrency ETF Selection Factors
Factor | Impact on ETF |
---|---|
Market Liquidity | Ensures ease of trading and reduces slippage |
Volatility | Helps AI to balance high-risk and low-risk assets |
Technology Assessment | Identifies promising projects with growth potential |
Sentiment Analysis | Anticipates price movements based on public mood |
How Artificial Intelligence Enhances Stock Prediction Accuracy in Cryptocurrency ETFs
The integration of artificial intelligence (AI) into cryptocurrency ETFs has significantly transformed the accuracy of stock predictions, providing investors with an advanced edge. AI models leverage vast datasets, including market sentiment, technical indicators, and historical trends, to identify patterns that human analysts might overlook. The ability to analyze data from multiple sources in real-time allows these models to deliver precise, data-driven forecasts, enhancing portfolio management strategies in crypto-focused ETFs.
AI algorithms continuously adapt to changing market conditions, improving prediction reliability. By incorporating machine learning techniques, these models can refine their predictions over time based on new data inputs. As a result, ETFs with AI-driven stock selection can outperform traditional methods, especially in the volatile cryptocurrency market, where price fluctuations are often unpredictable and influenced by a variety of global factors.
How AI Algorithms Improve Prediction Accuracy
- Data-Driven Insights: AI models can process and analyze vast amounts of structured and unstructured data, offering a more comprehensive view of the market.
- Pattern Recognition: Machine learning algorithms excel in detecting intricate patterns within complex data sets, even in highly volatile markets like cryptocurrency.
- Real-Time Adaptability: AI models are capable of adjusting predictions in real-time based on new market data, enhancing forecast accuracy.
- Risk Mitigation: By recognizing potential market downturns, AI can suggest timely adjustments to portfolios, reducing exposure to losses.
AI also plays a crucial role in sentiment analysis, which is essential for predicting market movements in cryptocurrency. AI models analyze social media, news articles, and online forums to gauge public sentiment, a key factor influencing cryptocurrency prices.
Key Benefit: The ability to assess public sentiment gives AI models an edge in predicting sudden shifts in market trends, which is especially valuable in the unpredictable crypto market.
Examples of AI-Enhanced Cryptocurrency ETFs
ETF Name | AI Model Used | AI Impact |
---|---|---|
Crypto AI ETF | Neural Networks | Improved prediction accuracy by 15% in volatile market conditions. |
Blockchain Predictive ETF | Deep Learning | Enhanced portfolio performance by adapting to real-time data fluctuations. |
Advantages of AI-Powered ETFs Over Conventional Stock Selection Strategies
AI-based exchange-traded funds (ETFs) are quickly becoming a key player in the investment landscape, offering several advantages over traditional stock picking methods, particularly in the context of the cryptocurrency market. While traditional methods rely heavily on human expertise and emotional decision-making, AI-driven ETFs leverage sophisticated algorithms to process vast amounts of data, identifying patterns and trends that human investors might overlook. This results in more efficient, data-driven investment strategies that can outperform conventional approaches, especially in highly volatile sectors like cryptocurrencies.
Unlike traditional stock pickers who may rely on historical trends, news, or intuition, AI systems can instantly process and react to real-time market movements, offering the potential for better returns with reduced risk. The adoption of AI in ETFs ensures that investment decisions are backed by cutting-edge technology, minimizing the biases and errors associated with human judgment. This capability is particularly valuable in the world of cryptocurrencies, where market shifts can be rapid and unpredictable.
Key Advantages of AI-Based ETFs
- Data-Driven Decision Making: AI systems continuously analyze vast datasets, identifying market trends, correlations, and potential investment opportunities that humans might miss.
- 24/7 Monitoring: AI can monitor cryptocurrency markets around the clock, reacting to sudden price changes or news events more quickly than human traders.
- Reduced Emotional Bias: Unlike human investors, AI does not react emotionally to market fluctuations, providing more consistent and objective decisions.
- Scalability: AI can process much larger datasets than a human investor, improving its ability to make predictions across multiple markets and assets simultaneously.
Traditional Methods vs. AI ETFs
Aspect | Traditional Stock Picking | AI-Based ETFs |
---|---|---|
Speed | Slow decision-making based on human analysis | Real-time decision-making with automated responses |
Emotional Influence | Decisions may be influenced by human emotions (fear, greed) | Decisions based solely on data analysis, free from emotions |
Data Processing | Limited to the analysis that a human can process | Capable of processing vast amounts of data instantly |
AI-based ETFs offer a more systematic and efficient approach to investing, particularly in the highly volatile cryptocurrency space, where market shifts can happen within minutes. With its data-driven decision-making capabilities, AI can provide a strategic edge over traditional stock picking techniques.
Evaluating the Effectiveness of an AI-Driven Cryptocurrency ETF
When assessing the performance of a cryptocurrency ETF powered by artificial intelligence, it is crucial to consider various factors that differentiate it from traditional investment vehicles. AI-driven ETFs are designed to leverage machine learning algorithms to analyze vast amounts of market data, identify trends, and make informed decisions about which cryptocurrencies to include in the portfolio. However, understanding how well these ETFs perform requires a systematic approach that goes beyond simple price movement analysis.
To accurately evaluate the effectiveness of an AI-powered crypto ETF, investors should look at the following key metrics and evaluation methods:
Key Metrics for Evaluation
- Volatility: AI algorithms must account for the extreme volatility often seen in the cryptocurrency market. Assessing how well an AI ETF manages this volatility is essential to understanding its risk profile.
- Returns Comparison: Compare the ETF's performance against both the cryptocurrency market index and other AI-driven investment products to gauge relative success.
- Adaptability: The AI model's ability to adapt to changing market conditions and adjust its holdings accordingly is critical for long-term performance.
- Transparency: Evaluate the level of transparency the ETF provider offers regarding the AI model's decision-making process and the data it uses.
Performance Analysis Tools
- Backtesting: Running historical simulations to see how the AI-driven ETF would have performed during various market conditions can provide valuable insights into its potential future performance.
- Sharpe Ratio: This metric helps assess the risk-adjusted return of the ETF, which is crucial in evaluating its overall profitability relative to the risk taken.
- Tracking Error: The tracking error measures the deviation of the ETF’s returns from the benchmark cryptocurrency index, helping to understand the accuracy of its stock selection and strategy.
It’s important to note that while AI can make data-driven decisions, the unpredictable nature of cryptocurrencies means that past performance is not always indicative of future results.
Sample Performance Table
Metric | AI ETF | Market Index |
---|---|---|
Annual Return | 25% | 20% |
Volatility | 30% | 35% |
Sharpe Ratio | 1.2 | 0.8 |
Common Risks of AI-Based ETFs in Cryptocurrency and Their Mitigation Strategies
Investing in AI-powered ETFs that focus on cryptocurrency carries inherent risks due to the volatile nature of the crypto market and the reliance on algorithms for stock selection. These funds often use machine learning models to predict price trends and select digital assets, but their performance is heavily dependent on the accuracy and adaptability of these models. A poorly designed or overfitted algorithm can lead to significant financial losses, particularly when market conditions change abruptly.
Additionally, AI-driven ETFs might overlook crucial market factors such as investor sentiment or sudden regulatory changes, which are common in the cryptocurrency space. This reliance on data can also lead to systemic risks where the ETF behaves similarly to other funds, creating potential for larger-scale losses in a market downturn. To minimize these risks, it's essential to adopt proper risk management techniques and diversify holdings.
Key Risks and How to Mitigate Them
- Market Volatility: Cryptocurrencies are highly volatile, and AI algorithms might misinterpret sudden shifts in market conditions.
- Model Overfitting: AI models that perform well in backtesting may fail in real-world conditions due to overfitting to historical data.
- Data Quality: The accuracy of AI predictions heavily relies on the quality of data fed into the model. Inaccurate or incomplete data can lead to poor investment decisions.
- Regulatory Risks: Changes in cryptocurrency regulations could negatively impact the performance of AI-driven ETFs that are heavily invested in digital assets.
Risk Management Strategies
- Diversification: Avoid concentration in a single asset class. Invest in a variety of cryptocurrencies or other asset types to reduce exposure to market downturns.
- Regular Model Evaluation: Continuously assess the performance of the AI model to ensure it remains effective in changing market conditions.
- Use of Stop-Loss Mechanisms: Set automated stop-loss orders to minimize potential losses during extreme market swings.
- Stay Updated on Regulatory Changes: Regularly monitor the regulatory landscape for cryptocurrencies and adjust your ETF exposure accordingly.
Important: AI-driven ETFs in the cryptocurrency space are not immune to the same risks as other financial products. Always ensure you conduct thorough research before investing.
Example of Risk Factors Table
Risk Factor | Impact | Mitigation Strategy |
---|---|---|
Market Volatility | High fluctuations in crypto prices can lead to unexpected losses | Diversification and setting stop-loss limits |
Model Overfitting | Algorithms may fail to adapt to changing market conditions | Regular model testing and adjustments |
Data Quality | Inaccurate data may skew investment decisions | Invest in high-quality, verified data sources |
Regulatory Risks | Changes in law can negatively impact investments | Stay informed on regulatory changes and adapt investment strategy |