Ai Driven Options Trading
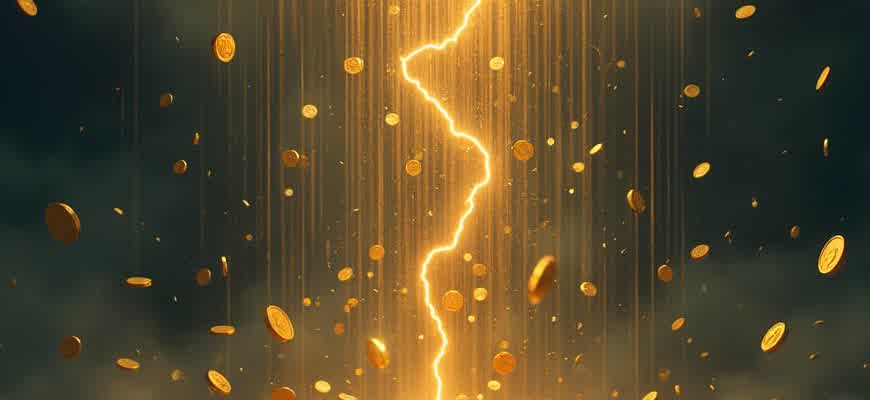
Recent advancements in artificial intelligence (AI) have transformed various sectors of finance, including cryptocurrency options trading. With the ability to process massive amounts of data and make real-time decisions, AI-powered systems are becoming a crucial tool for traders aiming to optimize their strategies in the volatile world of cryptocurrencies.
AI models leverage machine learning algorithms to predict market movements and identify optimal entry and exit points for trades. Unlike traditional methods, these systems can continuously adapt to changing market conditions, making them highly effective in environments as dynamic and unpredictable as the crypto market.
- Data-Driven Analysis: AI utilizes vast datasets, including historical price movements, trading volume, and sentiment analysis from social media and news sources, to make informed decisions.
- Real-Time Decision Making: AI algorithms can react instantly to market shifts, offering traders an edge in executing timely trades.
- Risk Management: AI systems are designed to assess risks based on multiple variables, which helps minimize potential losses during high-volatility periods.
Key Benefits of AI in Crypto Options Trading:
Benefit | Description |
---|---|
Speed | AI systems process data and execute trades much faster than human traders, reducing the chances of missed opportunities. |
Accuracy | Machine learning models can analyze patterns more accurately, leading to better predictions and fewer errors. |
Scalability | AI-driven platforms can handle large volumes of trades and data simultaneously, enabling scalability without sacrificing performance. |
AI-driven trading strategies have the potential to revolutionize the cryptocurrency market by eliminating human error and leveraging computational power to make data-backed, objective decisions.
AI-Driven Crypto Options Trading: A Complete Guide
The landscape of cryptocurrency trading has evolved rapidly, with artificial intelligence (AI) playing a pivotal role in reshaping how investors approach options trading. AI-powered systems can analyze vast amounts of market data, detect trends, and predict price movements, offering traders a distinct advantage in the volatile crypto markets. By integrating machine learning algorithms and predictive analytics, AI is transforming the way options contracts are priced, traded, and managed, especially in highly speculative environments like cryptocurrency.
This guide delves into the mechanics of AI-driven options trading within the realm of cryptocurrencies, exploring the key components and strategies that traders can utilize. From automated strategies to real-time decision-making, AI technologies enable crypto traders to respond to market changes swiftly and with precision, thereby enhancing the potential for profit while mitigating risk.
Key Features of AI in Crypto Options Trading
- Predictive Analytics: AI models use historical data, news sentiment, and market signals to forecast future price movements of cryptocurrencies. This allows for more accurate predictions of options pricing.
- Automated Trading Systems: AI-driven platforms can execute trades automatically based on predefined criteria, ensuring timely actions without human intervention.
- Risk Management: Advanced algorithms monitor market conditions and adjust trading strategies in real-time to minimize potential losses while maximizing profits.
How AI Enhances Decision-Making in Crypto Options
- Data Collection and Analysis: AI systems gather vast amounts of real-time and historical data from multiple crypto exchanges to provide insights into market sentiment and volatility.
- Pattern Recognition: Machine learning models identify recurring price patterns and correlations, enabling traders to predict market shifts more effectively.
- Strategy Optimization: AI continuously refines trading strategies by evaluating past trades and adjusting for better outcomes in future transactions.
"AI-powered systems don't just react to the market, they learn from it, adapting to new information faster than any human trader could. This ability to evolve in real-time makes them especially useful in the fast-paced world of cryptocurrency options trading."
Table: AI vs. Traditional Trading in Crypto Options
Aspect | AI-Driven Trading | Traditional Trading |
---|---|---|
Speed of Execution | Milliseconds | Seconds to minutes |
Data Processing | Real-time analysis of large datasets | Manual data review |
Market Adaptability | Adapts in real-time to new data | Changes based on human judgment and experience |
Risk Management | Dynamic and automated risk control | Dependent on trader's discretion |
Understanding AI Algorithms in Cryptocurrency Options Trading
In the rapidly evolving cryptocurrency market, artificial intelligence (AI) has become a key player in enhancing options trading strategies. By leveraging machine learning and deep learning algorithms, AI is capable of analyzing vast amounts of data, identifying patterns, and making real-time predictions that would be impossible for human traders to process manually. This ability to sift through massive datasets gives traders an edge in making quicker, more accurate decisions in a highly volatile environment.
AI-driven strategies in cryptocurrency options trading are fundamentally different from traditional methods. They not only consider price movements but also integrate a wide array of factors like sentiment analysis, market news, and social media trends. As these algorithms continuously evolve, they can adapt to new market conditions, providing traders with the ability to optimize their strategies based on current and predicted market behavior.
Key Components of AI Algorithms in Trading
- Machine Learning Models: These models are designed to improve themselves over time, learning from past trades to make better future decisions. They can predict market movements based on historical data and trends.
- Sentiment Analysis: AI tools analyze online news, social media, and forums to gauge market sentiment, helping traders anticipate price shifts that may not be immediately visible in raw price data.
- Neural Networks: Complex deep learning models capable of recognizing intricate patterns and relationships in the data that human traders might miss, enhancing decision-making accuracy.
Algorithmic Trading vs Traditional Methods
Aspect | AI-Driven Trading | Traditional Trading |
---|---|---|
Speed | Instantaneous processing of large volumes of data | Manual analysis, slower decision-making |
Adaptability | Dynamic and self-optimizing models | Rigid strategies based on fixed parameters |
Data Utilization | Real-time data from multiple sources (price, sentiment, news) | Primarily based on historical price data |
Important Note: The real advantage of AI in options trading is its ability to process and act on data in real-time, allowing for rapid adjustments to strategy as market conditions evolve.
Challenges in AI-Driven Cryptocurrency Trading
- Data Quality: The effectiveness of AI algorithms is highly dependent on the quality and accuracy of the data they are trained on. Inaccurate or incomplete data can lead to suboptimal predictions.
- Market Volatility: While AI can process large amounts of data, sudden market shifts due to unexpected events can still challenge the algorithms, making them less reliable in certain extreme conditions.
- Overfitting: If not properly managed, AI models may overfit to historical data, resulting in poor performance in live trading situations.
How AI Predicts Cryptocurrency Market Movements for Derivative Trading
The application of AI in predicting cryptocurrency market movements has revolutionized options trading. Algorithms utilize vast datasets from various sources, including price trends, social media sentiment, and blockchain data, to model future price fluctuations. By processing this data in real-time, AI systems can identify patterns and correlations that are often imperceptible to human traders. This enables more precise predictions for both short-term price shifts and longer-term market trends.
Machine learning models, specifically neural networks, are particularly effective at analyzing the non-linear behaviors seen in cryptocurrency markets. These models can be trained to recognize patterns in the data, constantly refining their predictions as new information becomes available. In the case of options trading, AI is not just predicting price directions but also assessing volatility, liquidity, and the optimal timing for entering and exiting positions.
AI-Based Predictive Models for Crypto Derivative Markets
AI utilizes various methodologies to forecast market trends, especially in volatile sectors like cryptocurrencies:
- Sentiment Analysis: AI evaluates social media and news outlets to gauge public sentiment, which is crucial for volatile assets like Bitcoin and Ethereum.
- Time-Series Forecasting: Algorithms examine historical price data, identifying recurring patterns that can predict future price movements.
- Volatility Models: AI models assess market volatility to predict potential price swings, which is essential for options pricing.
Key Techniques for Predicting Cryptocurrency Price Movements
- Deep Learning: Neural networks are used to analyze complex relationships in cryptocurrency data, improving accuracy in price prediction.
- Reinforcement Learning: AI learns from the outcomes of previous trades, adapting its strategies to maximize profitability over time.
- Algorithmic Trading: Automated systems continuously monitor the market, executing trades based on the AI's real-time analysis.
AI not only predicts the direction of cryptocurrency price movement but also assesses the potential risks and rewards associated with options contracts, helping traders optimize their strategies.
Example of AI-Driven Prediction Results
Model | Prediction Accuracy | Trade Efficiency |
---|---|---|
Neural Network | 85% | High |
Sentiment Analysis | 80% | Moderate |
Time-Series Forecasting | 90% | Very High |
Selecting the Right AI Model for Cryptocurrency Trading
In the volatile world of cryptocurrency trading, selecting an appropriate AI model is crucial for optimizing strategies and increasing the chances of profitability. Different models offer varying levels of sophistication and predictive power, which is why understanding their strengths and weaknesses is essential for crafting effective trading systems. Factors such as market dynamics, data volume, and specific goals will all influence the choice of model.
When developing AI-driven strategies for crypto trading, it's important to consider the type of analysis you want to perform–whether it's trend prediction, volatility forecasting, or real-time decision making. Choosing the right model can mean the difference between achieving consistent profits and facing significant losses in this unpredictable market.
Factors to Consider When Choosing the Model
- Data Availability: Access to large and high-quality datasets is crucial for AI models to make accurate predictions. Models like LSTM (Long Short-Term Memory) networks perform well with time-series data, while reinforcement learning thrives on high-frequency trading data.
- Computational Power: Complex models, such as deep learning algorithms, require significant computational resources, making them suitable for high-frequency traders or institutions with advanced infrastructure.
- Risk Tolerance: Certain models can be more aggressive in their predictions, while others, like risk-adjusted algorithms, are designed to minimize losses. Tailoring the model to your risk profile ensures more appropriate decision-making in fast-moving markets.
Popular AI Models for Cryptocurrency Trading
- Recurrent Neural Networks (RNNs): Ideal for time-series forecasting, RNNs can process sequences of market data and predict future price movements based on historical trends.
- Reinforcement Learning (RL): In this model, AI agents learn by interacting with the market and receiving feedback based on their performance, enabling them to adapt and optimize trading strategies.
- Support Vector Machines (SVM): Often used for classification tasks, SVMs can be effective in identifying buy or sell signals by distinguishing between bullish and bearish market conditions.
"The success of your AI trading model is directly linked to how well you understand both the underlying technology and the market conditions it is designed to navigate."
Choosing Based on Strategy Type
Strategy Type | Recommended AI Model | Advantages |
---|---|---|
Trend Prediction | LSTM (Long Short-Term Memory) | Effective at forecasting long-term trends based on historical data |
Volatility Forecasting | GARCH Models | Good at modeling and predicting financial volatility, often used in high-frequency trading |
Real-Time Decision Making | Reinforcement Learning | Optimizes trading strategies through continuous learning from market interactions |
Setting Up Cryptocurrency AI-Driven Trading Systems: A Step-by-Step Guide
Implementing AI in cryptocurrency trading requires understanding both the technology and the dynamics of digital asset markets. With cryptocurrencies being volatile and decentralized, leveraging machine learning and advanced algorithms can enhance trading strategies and decision-making processes. AI models can quickly analyze large datasets and adjust to market fluctuations faster than traditional methods. However, setting up a successful AI-driven trading system involves careful planning and strategic steps.
The process begins with defining your objectives and understanding the crypto market's unique characteristics. Unlike traditional stocks, cryptocurrencies experience 24/7 trading, often requiring algorithms that can handle real-time data at any hour. It's essential to build a solid infrastructure to support your AI-driven system and ensure it can handle high-frequency data, perform rapid calculations, and adapt to market changes in real-time.
Step 1: Choosing the Right AI Framework
Before deploying AI into your trading strategy, you need to select an appropriate machine learning framework. Popular options include TensorFlow, PyTorch, and scikit-learn. The right choice will depend on your needs for deep learning, neural networks, and data processing capabilities.
- TensorFlow – Ideal for large-scale machine learning projects and deep neural networks.
- PyTorch – Best for research purposes and offers dynamic computation graphs.
- scikit-learn – A great tool for classical machine learning models such as regression, classification, and clustering.
Step 2: Data Collection and Preprocessing
AI models require large amounts of high-quality data to train effectively. In cryptocurrency trading, you will need to gather data from multiple sources such as price feeds, order book data, and market sentiment indicators. This data must be cleaned and preprocessed to ensure it’s structured correctly and free from noise.
- Historical price data: This is critical for understanding trends and building predictive models.
- Market depth and liquidity: Data about the order book will help the AI model to understand market sentiment and potential price manipulation.
- Sentiment analysis: Extracting sentiment from social media platforms like Twitter or news articles can provide additional context to price movements.
Step 3: Model Training and Evaluation
Once the data is prepared, the next step is training the model. This involves feeding the historical data into machine learning algorithms and adjusting the parameters to optimize predictive accuracy. Regular evaluation of the model’s performance is critical to avoid overfitting and ensure it generalizes well to unseen data.
It’s essential to split your dataset into training, validation, and test sets to avoid overfitting and ensure robust predictions.
Step 4: Implementing the Trading Strategy
After training the model, the next step is to integrate it into the trading system. A trading bot can execute buy and sell orders based on predictions made by the AI. This bot should be able to monitor market conditions, place trades, and adjust positions in real-time. It’s critical to incorporate risk management strategies like stop-loss and take-profit levels to minimize potential losses.
Strategy | Description |
---|---|
Trend Following | The AI bot looks for and follows market trends, buying when the market is bullish and selling when it turns bearish. |
Mean Reversion | The bot buys when prices fall below a certain threshold and sells when they rise above a set level. |
Arbitrage | Exploits price differences between different exchanges to buy low and sell high. |
By following these steps, you can build a robust AI-driven trading system tailored to the unique challenges of cryptocurrency markets. Proper testing and continuous monitoring will be key to maintaining performance and adjusting strategies in an ever-changing environment.
Optimizing AI Models for Better Accuracy in Predicting Cryptocurrency Option Prices
The growing influence of artificial intelligence (AI) in the world of cryptocurrency options trading is undeniable. As traders seek higher returns with lower risk, predicting option prices with precision is essential. AI models, when optimized correctly, can provide valuable insights by analyzing vast amounts of market data, uncovering hidden patterns, and making predictions with greater accuracy. However, ensuring the AI's performance in a volatile market like cryptocurrency requires careful adjustments to both model architecture and the data pipeline.
Improving the prediction accuracy for cryptocurrency options involves various strategies, from data preprocessing to selecting appropriate learning algorithms. Among the most important factors in optimizing AI for this purpose are feature engineering, model training techniques, and hyperparameter tuning. Each of these areas requires a deep understanding of both the market dynamics and AI capabilities to achieve a high level of precision in option price forecasts.
Key Approaches to Optimize AI Models
- Data Quality and Feature Engineering: The quality of input data is a major factor in the success of any AI model. In the case of cryptocurrency options, factors such as price volatility, trading volume, and market sentiment must be considered. Carefully selecting and transforming raw market data into meaningful features improves the model’s ability to identify critical patterns.
- Algorithm Selection: Depending on the complexity of the data, AI models like neural networks or reinforcement learning techniques may offer the best results. Deep learning approaches, particularly Long Short-Term Memory (LSTM) networks, are well-suited for sequential market data, while gradient boosting methods may perform better on smaller datasets with a lot of noise.
- Hyperparameter Tuning: The effectiveness of AI models can significantly improve through hyperparameter optimization. Techniques such as grid search or random search help identify the best parameters for optimal model performance, which is especially important for predicting highly volatile cryptocurrency options prices.
Important Tip: It is crucial to validate AI models regularly with out-of-sample data to ensure they remain effective in different market conditions, especially in the fast-moving cryptocurrency space.
Performance Metrics for AI-based Option Price Prediction
Metric | Description |
---|---|
Mean Absolute Error (MAE) | Measures the average absolute difference between predicted and actual option prices. |
Root Mean Squared Error (RMSE) | Measures the square root of the average squared differences between predicted and actual values, giving more weight to larger errors. |
R-Squared | Represents the proportion of the variance in the dependent variable (option price) that is predictable from the independent variables. |
Key Insight: To achieve consistently high prediction accuracy, it's essential to monitor not only the model's training performance but also its behavior on real-time data.
AI-Driven Risk Management in Crypto Options Trading
The volatile nature of cryptocurrency markets makes risk management a critical aspect of options trading. Traditional risk mitigation strategies often fall short due to the rapid and unpredictable price movements seen in digital assets. With AI-powered tools, traders can access more precise and dynamic approaches to managing risks in the highly speculative world of crypto options trading.
Artificial intelligence offers advanced predictive models, real-time data analysis, and automation to improve decision-making and reduce exposure to adverse market movements. By leveraging machine learning algorithms, traders can enhance their ability to anticipate price fluctuations, manage portfolio risks, and execute trades with greater confidence in an otherwise unpredictable environment.
Key Risk Management Strategies with AI
AI can be used to optimize various risk management techniques in cryptocurrency options trading:
- Dynamic Hedging: AI models can continuously assess market conditions and adjust hedging strategies in real time to limit potential losses.
- Volatility Forecasting: AI tools can analyze historical price data to predict short-term volatility, enabling traders to better anticipate price movements.
- Sentiment Analysis: AI-powered sentiment analysis tools can gauge the mood of the market by scanning social media and news sources, giving traders insight into upcoming price shifts.
Risk Mitigation through AI Algorithms
AI algorithms can improve decision-making by identifying patterns that may not be immediately obvious to human traders. A few examples include:
- Monte Carlo Simulations: AI models use these simulations to forecast potential market scenarios and estimate risk exposure under different conditions.
- Portfolio Optimization: AI tools can balance options positions and digital assets to ensure maximum returns with minimized risk.
- Stop-Loss Automation: AI can implement stop-loss orders automatically based on real-time data to protect against excessive losses.
Impact of AI on Risk-Adjusted Returns
AI's ability to continuously process vast amounts of data allows traders to respond swiftly to changing market conditions. This results in improved risk-adjusted returns and greater overall efficiency in managing a crypto options portfolio.
Risk Management Technique | AI Application | Benefit |
---|---|---|
Dynamic Hedging | Real-time risk assessment and adjustment | Limits losses in fluctuating markets |
Volatility Forecasting | Predictive models using historical data | Aids in anticipating price fluctuations |
Sentiment Analysis | Monitoring market sentiment through AI | Predicts market movements before they happen |
Important: By integrating AI into crypto options trading, traders gain the advantage of real-time analysis and automated decision-making, crucial for navigating the volatile nature of the digital asset market.