Ai Driven Crypto Trading
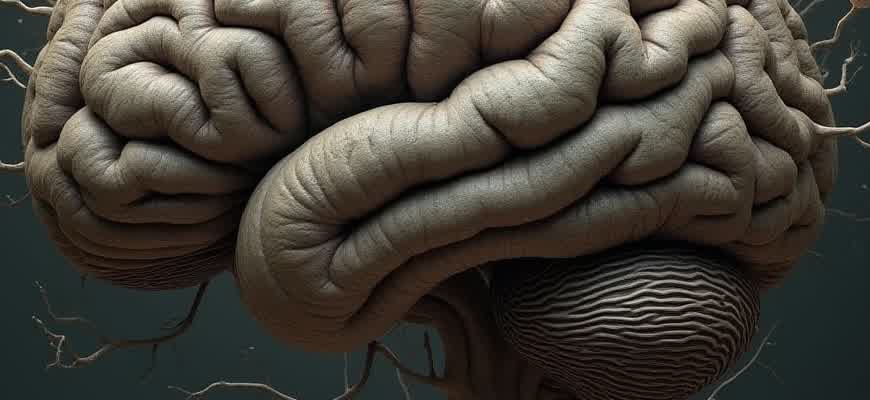
In recent years, artificial intelligence (AI) has revolutionized the world of cryptocurrency trading. By leveraging complex algorithms and machine learning, traders and investors can now make more informed, data-driven decisions in real time. This technological shift has made it possible to analyze vast amounts of market data quickly, identify patterns, and execute trades with minimal human intervention.
AI-driven trading platforms are designed to optimize trading strategies through continuous learning and adaptation. By utilizing predictive models and deep learning, these systems can assess market conditions, monitor price fluctuations, and react to external factors faster than traditional methods. The result is the ability to capture profits in a highly volatile market with improved accuracy.
- Real-time data analysis
- Automated decision-making
- Adaptive learning algorithms
Below is a comparison of key features of AI-driven trading platforms versus traditional manual trading methods:
Feature | AI-Driven Trading | Traditional Trading |
---|---|---|
Speed of Execution | Milliseconds | Seconds/Minutes |
Market Analysis | Automated, based on large datasets | Human-driven, often based on intuition |
Risk Management | AI-powered risk models | Manual risk assessment |
"AI in cryptocurrency trading is not just about automation–it's about creating systems that can learn, adapt, and ultimately outperform human traders."
AI-Powered Cryptocurrency Trading: Leveraging Technology for Enhanced Profits
As the cryptocurrency market continues to grow in complexity and volatility, traditional methods of trading are often insufficient to keep up. Traders are increasingly turning to artificial intelligence (AI) to gain an edge. AI-driven strategies not only analyze vast amounts of data in real-time but also predict market trends with greater accuracy, offering a powerful tool for maximizing profit potential. By incorporating machine learning, neural networks, and natural language processing, these technologies can adapt and optimize trading decisions far faster than any human trader ever could.
AI systems excel in pattern recognition, sentiment analysis, and automated decision-making, which are essential for navigating the ever-changing crypto landscape. Traders can use AI to identify optimal entry and exit points, spot emerging market trends, and reduce the risks associated with emotional or impulsive trading decisions. Below is an overview of how AI enhances profitability in crypto trading.
Key Benefits of AI in Crypto Trading
- Real-Time Data Processing: AI can analyze enormous volumes of real-time data, including market fluctuations, news, and social media sentiment, providing traders with timely insights.
- Predictive Analytics: AI uses historical data and machine learning algorithms to forecast price movements, identifying profitable opportunities before they become obvious to the broader market.
- Emotion-Free Trading: AI removes human emotions from trading decisions, ensuring that trades are executed purely based on data and algorithms.
- 24/7 Trading: AI bots can operate continuously, providing traders with the ability to take advantage of market shifts, even when they are not actively monitoring the market.
"The speed and efficiency of AI-driven trading can significantly outperform human traders, leading to more consistent profits and reduced risk."
AI Tools Used in Crypto Trading
- Algorithmic Trading Bots: These bots follow predefined sets of rules, executing trades automatically based on real-time data.
- Machine Learning Models: Models that learn from historical data to predict future market trends and adjust strategies accordingly.
- Sentiment Analysis Tools: Tools that gauge market sentiment by analyzing news articles, social media, and other sources of public opinion to anticipate price movements.
AI-Driven Trading Performance Comparison
Method | Efficiency | Risk Level | Time Spent |
---|---|---|---|
AI-Driven Trading | High | Low | Minimal |
Manual Trading | Medium | High | High |
Algorithmic Trading | High | Medium | Low |
How AI Models Predict Cryptocurrency Price Movements
The prediction of cryptocurrency prices through artificial intelligence (AI) is one of the most promising advancements in the digital finance space. By leveraging advanced algorithms, AI systems analyze massive datasets in real-time, identify patterns, and generate forecasts based on historical market data. Machine learning models, such as supervised learning, reinforcement learning, and deep learning, are the primary tools used to predict price fluctuations. These models are particularly adept at processing non-linear data and accounting for market sentiment, which traditional models often struggle to interpret.
AI models utilize a wide variety of techniques to forecast cryptocurrency trends. By incorporating both structured and unstructured data, such as market prices, trading volumes, social media sentiment, and even news headlines, these algorithms can predict price movements with impressive accuracy. However, the highly volatile nature of crypto markets makes predictions inherently uncertain. Nonetheless, AI offers a significant advantage by continuously adapting to new data, improving accuracy over time.
AI Techniques in Cryptocurrency Price Forecasting
- Time Series Analysis: AI uses historical price data to predict future trends. Time series models like ARIMA and LSTM networks are particularly effective for this purpose.
- Sentiment Analysis: By analyzing social media posts, news articles, and forums, AI models can assess market sentiment and predict its potential influence on cryptocurrency prices.
- Reinforcement Learning: AI models learn to make decisions by interacting with the market environment, adjusting strategies based on past outcomes.
"AI-powered models not only analyze numerical data but also integrate sentiment and behavioral patterns to create more holistic predictions, which is key in the volatile crypto markets."
AI Models' Approach to Data Collection
- Price Data: Historical price trends, trading volume, and market liquidity are essential inputs for most predictive models.
- Social Media & News: Real-time sentiment from Twitter, Reddit, and financial news outlets can provide early indications of market movements.
- Blockchain Data: AI can also analyze on-chain data, including transaction volumes, wallet balances, and exchange inflows/outflows, to gauge investor behavior.
Example of AI Price Prediction Accuracy
Model | Prediction Accuracy | Use Case |
---|---|---|
ARIMA | 80-85% | Short-term price prediction |
LSTM (Deep Learning) | 85-90% | Long-term trend forecasting |
Sentiment Analysis | 75-80% | Market sentiment-driven price change prediction |
Choosing the Right AI-Powered Crypto Trading Platform for Investments
As the cryptocurrency market becomes increasingly volatile, utilizing artificial intelligence (AI) in trading has gained significant attention. AI-based trading platforms can help investors make more informed decisions by analyzing massive datasets in real time, detecting trends, and executing trades at optimal times. However, with the growing number of AI-driven crypto platforms, selecting the right one can be a challenging task. Understanding the key factors to consider can help investors navigate this complex landscape.
When choosing an AI crypto trading platform, there are several critical elements to evaluate, such as the platform's algorithmic trading capabilities, user interface, security measures, and cost structures. These features will directly influence the efficiency and profitability of your trading strategy. Below are some essential aspects to consider when selecting an AI-driven platform for cryptocurrency investments.
Key Features to Look For
- Algorithm Accuracy: The quality of AI algorithms and their ability to adapt to market changes is vital for successful crypto trading.
- Security Protocols: Ensure the platform uses robust security measures such as two-factor authentication (2FA) and encryption to protect your funds and personal information.
- Backtesting Tools: Platforms offering backtesting features allow users to simulate trading strategies based on historical data, helping to evaluate their effectiveness.
- User Interface: A clean, intuitive interface enhances the trading experience, especially for those new to crypto trading.
- Cost and Fees: Review the platform's fee structure–whether it's based on the number of trades, assets under management, or performance-related fees–to ensure it aligns with your investment strategy.
Top Platforms Comparison
Platform | Algorithm Precision | Security | Fees |
---|---|---|---|
Platform A | High | End-to-end Encryption, 2FA | 0.5% per trade |
Platform B | Medium | Cold Wallet Storage | 1% per trade |
Platform C | Very High | Insurance Fund, 2FA | Performance-Based |
“The key to successful AI trading is not only relying on the platform's algorithms but also understanding your own risk tolerance and setting appropriate parameters for the AI to operate within.”
Additional Considerations
- Market Integration: Check if the platform supports integration with multiple cryptocurrency exchanges for better liquidity.
- Customer Support: Ensure there’s a responsive customer support team available to assist with any technical or account-related issues.
- Real-Time Analytics: Platforms offering live market data analysis and AI-generated insights can provide an edge in making informed decisions quickly.
Key Algorithms Behind AI-Driven Crypto Trading Strategies
The integration of AI into cryptocurrency trading strategies has brought about a new era of automated decision-making, enhancing both speed and accuracy. Machine learning models, deep learning networks, and reinforcement learning techniques are leveraged to predict price movements and execute trades with minimal human intervention. These algorithms utilize vast amounts of historical data, real-time market analysis, and technical indicators to forecast trends, manage risk, and identify profitable opportunities in a volatile market. Below are some of the key algorithms that are commonly used in this space.
AI-driven trading platforms employ sophisticated models to assess market dynamics, leveraging both supervised and unsupervised learning techniques. The combination of these approaches allows traders to process a diverse range of inputs, including price history, volume, sentiment analysis, and news sentiment. In the following sections, we will look into the most widely used algorithms and their role in crypto trading.
1. Machine Learning Algorithms
- Supervised Learning - Trains models using labeled data, such as historical market data, to predict future price movements or asset performance.
- Unsupervised Learning - Identifies hidden patterns in the data without predefined labels, which is helpful for anomaly detection or clustering similar market behaviors.
- Deep Learning - Uses multi-layered neural networks for complex pattern recognition, particularly useful for processing vast datasets with intricate relationships.
2. Reinforcement Learning Algorithms
- Q-Learning - A model-free reinforcement learning algorithm used to determine the optimal trading strategy by learning from market actions and their consequences.
- Deep Q-Networks (DQN) - Combines deep learning with Q-learning to handle large state spaces, improving the trading model’s ability to make decisions in volatile environments.
3. Sentiment Analysis Algorithms
Another crucial component of AI trading strategies is sentiment analysis, which involves the use of natural language processing (NLP) to assess market sentiment based on news articles, social media posts, or tweets. These algorithms evaluate public opinion and social media trends to predict market shifts, incorporating both positive and negative sentiment signals into trading decisions.
Algorithm | Use Case | Key Advantage |
---|---|---|
Supervised Learning | Price prediction and trend analysis | High accuracy with historical data |
Reinforcement Learning | Dynamic strategy optimization | Self-improvement through market feedback |
Sentiment Analysis | Market sentiment prediction | Incorporates real-time public opinion |
Note: AI-driven trading systems are not foolproof and should be used alongside traditional risk management strategies. Despite their potential, they still face challenges such as overfitting and reliance on historical data that may not always predict future market movements accurately.
How Machine Learning Enhances Risk Management in Crypto Trading
In the highly volatile world of cryptocurrency trading, minimizing risks is paramount for both individual traders and institutions. Machine learning (ML) offers a sophisticated approach to identifying patterns and predicting market fluctuations, enabling traders to make more informed decisions. By leveraging large datasets and advanced algorithms, ML models can detect trends that would otherwise go unnoticed by human analysts, helping to anticipate price changes and mitigate the risks associated with market uncertainty.
Through techniques like supervised learning, unsupervised learning, and reinforcement learning, machine learning systems can adapt to real-time data, improving their predictions over time. This adaptability is crucial for dealing with the ever-changing dynamics of the crypto market. By reducing human error and automating decision-making processes, ML enhances the accuracy of risk management strategies and ensures more reliable trading outcomes.
How Machine Learning Reduces Risk in Crypto Trading
- Predictive Analytics: ML algorithms use historical price data, trading volume, and market sentiment to forecast potential price movements. This predictive power helps traders anticipate market trends and minimize the risk of sudden price drops.
- Sentiment Analysis: ML models can process social media feeds, news articles, and online forums to gauge public sentiment. By understanding the emotions driving market behavior, these models provide insights into market psychology, helping traders avoid panic-driven decisions.
- Portfolio Optimization: ML helps in optimizing crypto portfolios by balancing risk and return through advanced models that evaluate asset correlations, volatility, and other financial metrics.
Machine Learning Risk Management Techniques
- Volatility Forecasting: Predicting periods of high volatility allows traders to adjust their positions and reduce exposure during risky market conditions.
- Risk-Adjusted Returns: ML models help traders optimize their strategies to maximize returns while maintaining an acceptable level of risk.
- Anomaly Detection: Algorithms can identify irregular trading patterns or potential market manipulation, providing early warnings to traders.
Machine learning not only aids in predicting market movements but also provides traders with tools to automate risk management, allowing for quicker responses to unforeseen market shifts.
Technique | Benefit |
---|---|
Predictive Analytics | Anticipates market trends to make informed decisions. |
Sentiment Analysis | Identifies public sentiment to predict market movements. |
Portfolio Optimization | Improves risk-return balance in trading strategies. |
Integrating AI with Real-Time Market Data for Smarter Trades
AI-powered algorithms are transforming the landscape of cryptocurrency trading by integrating real-time market data. With such integration, traders can make decisions based on up-to-the-minute information, ensuring they capitalize on market fluctuations as soon as they happen. This approach contrasts with traditional methods, which often rely on delayed data and static analysis, leaving traders vulnerable to rapidly changing market conditions.
The combination of AI and real-time data allows for enhanced decision-making processes. Through machine learning models, AI can identify patterns, make predictions, and execute trades faster and more accurately than human traders. In essence, AI optimizes trading strategies by continuously learning from new data, making each trade smarter than the last.
Key Benefits of Real-Time Data and AI Integration
- Faster Execution: AI-driven systems can process vast amounts of market data instantly, allowing for faster decision-making and order execution.
- Continuous Learning: Machine learning models adapt and improve over time by learning from incoming data, increasing their accuracy in predicting price movements.
- Increased Accuracy: By using historical data, news sentiment analysis, and social media trends in real-time, AI algorithms can refine trading strategies to maximize returns.
"AI’s ability to analyze and act on real-time data opens up new possibilities for traders, ensuring they can respond to market shifts faster than ever before."
How AI Enhances Market Analysis
AI enhances market analysis by processing and analyzing different types of data in real time, such as price trends, order books, and news sentiment. This allows traders to spot opportunities that would be difficult to identify manually. AI can handle both structured and unstructured data, enabling a more comprehensive market overview.
For instance, by analyzing sentiment from social media platforms and crypto-related news sites, AI can predict how market sentiment might impact prices. Combined with price chart analysis, this can lead to a more accurate understanding of future price movements.
Example of AI-Driven Strategy
AI Model Type | Data Analyzed | Action Taken |
---|---|---|
Deep Learning Neural Network | Historical Prices, Market Volume, News Sentiment | Predicts Bullish Trend, Places Buy Orders |
Reinforcement Learning | Real-Time Market Data, Order Book | Adapts Strategy Based on Trade Outcomes |
The Role of Sentiment Analysis in AI-Driven Crypto Decision Making
In the fast-paced world of cryptocurrency trading, AI tools have become indispensable for making timely and informed decisions. Among these, sentiment analysis plays a crucial role by interpreting market sentiment from various data sources like news articles, social media, and forums. By evaluating the mood of the market–whether optimistic or pessimistic–AI systems can predict price movements and guide traders in making strategic decisions. This analysis allows algorithms to incorporate human psychology into trading strategies, an aspect that is essential in such volatile markets.
Traders often face challenges in determining the impact of market sentiment on price trends. AI models that utilize sentiment analysis help to solve this by processing vast amounts of textual data, identifying key trends and correlating them with market behaviors. As a result, these systems provide valuable insights, enabling both automated bots and human traders to capitalize on emerging opportunities or avoid risks before they materialize.
How Sentiment Influences Trading Decisions
Sentiment analysis uses natural language processing (NLP) algorithms to analyze public opinions and sentiments related to a cryptocurrency. By aggregating and interpreting data from multiple sources, AI can track the emotional trends surrounding a specific asset. This information is crucial for traders to forecast market shifts, which are often driven by emotions like fear, greed, or excitement.
- Market Sentiment Evaluation: AI identifies the collective mood of traders and investors, helping systems determine whether a coin is likely to experience upward or downward momentum.
- Real-Time Monitoring: AI can track and analyze sentiment in real time, ensuring that traders receive timely alerts and insights to adjust their strategies quickly.
- Predictive Analytics: By combining sentiment with other data points, AI models can predict future price movements and identify potential entry or exit points for traders.
Key Data Sources for Sentiment Analysis
- Social Media: Platforms like Twitter, Reddit, and Telegram are rich in user-generated content and discussions, providing valuable sentiment data.
- News Articles: Positive or negative headlines about a particular cryptocurrency can significantly impact its price.
- Forums and Blogs: Community-driven insights and opinions help reflect the overall market sentiment towards a specific cryptocurrency.
Example of Sentiment Analysis Impact
Data Source | Sentiment Detected | Expected Market Impact |
---|---|---|
Positive Sentiment | Potential Price Surge | |
Negative Sentiment | Likely Price Decline | |
News Headline | Neutral Sentiment | Stagnant Market Behavior |
"Sentiment analysis can drastically improve decision-making by anticipating market reactions based on emotional triggers, providing traders with a distinct advantage in volatile conditions."
Understanding Backtesting: How AI Simulates Crypto Trading Scenarios
Backtesting is a crucial process for evaluating trading strategies in the cryptocurrency market. It involves testing a model or algorithm on historical data to understand how it would have performed in past market conditions. AI-driven backtesting systems offer a more advanced approach, allowing for more accurate and dynamic simulations compared to traditional methods. By leveraging AI, traders can simulate a wide range of market scenarios and optimize their strategies based on performance metrics such as profitability, risk, and volatility.
The AI's ability to learn from past data and adjust its strategy over time makes backtesting particularly valuable in the highly volatile crypto space. Unlike conventional methods, AI can quickly analyze vast amounts of data, identify patterns, and even adjust for market anomalies. The results of AI-driven backtesting provide actionable insights that help traders make informed decisions, potentially leading to improved long-term performance.
AI Backtesting Workflow
AI backtesting follows a systematic process to simulate crypto trading scenarios. This includes data collection, strategy formulation, testing, and performance evaluation. Below is an overview of the workflow:
- Data Gathering: Collect historical price data, transaction volumes, and other relevant indicators.
- Strategy Design: Define the algorithm and parameters for the trading strategy.
- Simulation: The AI runs simulations based on historical data to test the strategy's performance.
- Performance Analysis: Evaluate the outcomes using metrics such as returns, drawdown, and Sharpe ratio.
Important Factors in AI Backtesting
During the backtesting process, several key factors influence the success of AI-driven simulations. These include:
- Market Volatility: Crypto markets are notoriously volatile, and AI must be able to account for this unpredictability.
- Data Accuracy: The quality of historical data used in backtesting directly affects the reliability of the results.
- Overfitting Risk: AI models should be cautious of overfitting to historical data, which could reduce their ability to adapt to future market conditions.
Key Takeaway: AI-driven backtesting enables traders to create more sophisticated models that can adapt to changing market conditions, offering a significant advantage in the fast-paced world of cryptocurrency trading.
Performance Metrics in AI Backtesting
When evaluating an AI model’s performance, several important metrics come into play. These allow traders to assess how well the strategy would have performed under various scenarios:
Metric | Definition |
---|---|
Return on Investment (ROI) | Measures the percentage gain or loss of the strategy over a period of time. |
Drawdown | The peak-to-trough decline during a specific period, indicating risk exposure. |
Sharpe Ratio | Assesses the risk-adjusted return by comparing excess returns to the standard deviation of returns. |