Best Ai Tools for Corporate Finance
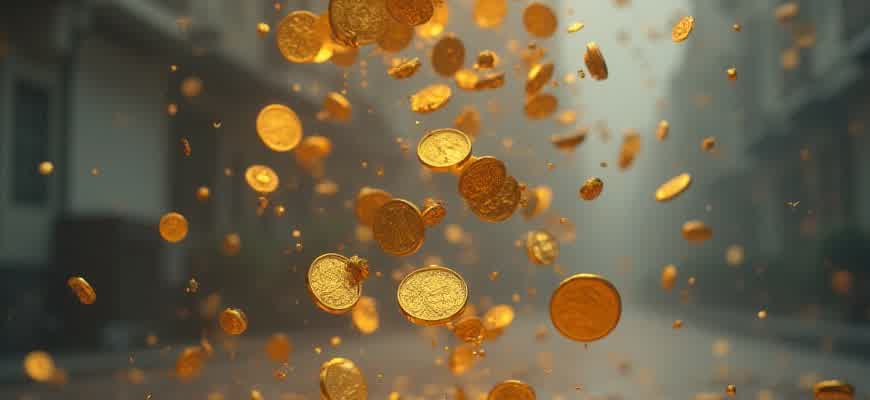
Adoption of intelligent systems within blockchain-driven businesses is reshaping how corporate finance departments handle forecasting, budgeting, and risk modeling. The following artificial intelligence tools offer powerful capabilities for decentralized companies seeking precision, automation, and scalable insights in financial operations.
- Numerai Signals – Uses encrypted data science models for hedge fund predictions, enabling crypto firms to access crowdsourced AI strategies.
- Domino Data Lab – Provides reproducible modeling environments ideal for building deep financial simulations in tokenized economies.
- Kensho – Specializes in real-time event detection and impact analysis, helping crypto treasuries adapt to global economic shifts.
Integrating AI into decentralized financial operations can reduce error margins by over 35% in budget planning and increase forecasting speed up to 50%.
For a structured comparison, here’s how these platforms align across key functions relevant to crypto-based financial workflows:
Tool | Forecast Accuracy | DeFi Integration | Risk Management |
---|---|---|---|
Numerai Signals | High | Moderate | Strong |
Domino Data Lab | Very High | High | Moderate |
Kensho | Moderate | Low | Very High |
Smart Solutions for Blockchain-Driven Corporate Finance
Integrating artificial intelligence into cryptocurrency-based finance allows companies to manage decentralized assets, forecast token value trends, and detect anomalies in real-time. AI algorithms are revolutionizing how treasuries handle digital assets, minimizing risks associated with volatility and regulatory complexity.
Financial departments leveraging blockchain ecosystems require tailored analytical tools to manage tokenized balance sheets, DeFi portfolios, and multi-chain treasury operations. These solutions offer strategic automation across liquidity forecasting, tax compliance, and crypto transaction auditing.
Leading Intelligent Platforms for Crypto-Centric Finance Teams
- Coin Metrics Atlas: AI-enhanced data infrastructure for institutional investors tracking blockchain flows and on-chain metrics.
- Covalent AI: Unified data layer enabling deep portfolio insights and anomaly detection across multiple chains.
- Chainalysis KYT: Machine learning for real-time compliance and monitoring of crypto transactions.
AI-powered blockchain analytics can reduce risk exposure by up to 45% in volatile market environments.
- Import multi-chain data through automated APIs.
- Run predictive algorithms to forecast asset performance.
- Generate compliance-ready reports for auditors and regulators.
Tool | Key Feature | Best For |
---|---|---|
Coin Metrics Atlas | Macro-level blockchain data | Strategy teams |
Covalent AI | Real-time portfolio monitoring | Corporate treasuries |
Chainalysis KYT | Transaction risk scoring | Compliance officers |
AI-Enhanced Forecasting Models in the Crypto Sector
In the rapidly evolving world of digital assets, predictive modeling powered by artificial intelligence is transforming how firms manage volatility and liquidity. These AI-based solutions digest vast on-chain and market data to project asset flows, token valuations, and risk exposure with unmatched precision. For treasury departments in crypto-native enterprises, these models are indispensable tools for preemptive financial strategy.
Unlike traditional static models, AI engines continuously learn from real-time crypto market behavior–incorporating shifts in trading volumes, whale movements, and protocol updates into forecasts. This dynamic learning capability reduces forecasting error and enhances capital efficiency across decentralized and centralized ecosystems.
Key Capabilities of AI in Crypto Forecasting
- On-Chain Behavior Analysis: Detects transaction patterns and liquidity movements in smart contracts.
- Sentiment Data Integration: Analyzes news and social media for early signals of market shifts.
- Volatility Pattern Recognition: Learns from historical price swings to predict future spikes or drops.
AI models adapt to the unique non-linear dynamics of the crypto markets, offering a strategic edge in managing treasury operations.
- Gather historical blockchain and exchange data.
- Apply neural time-series models (e.g., LSTM, GRU).
- Train on market anomalies and token-specific behaviors.
- Generate probabilistic scenarios for portfolio planning.
Feature | AI Model | Use Case |
---|---|---|
DeFi protocol flow forecasting | Reinforcement Learning | Yield optimization in staking strategies |
Token price prediction | LSTM Networks | Arbitrage and hedging decisions |
Wallet behavior analysis | Clustering Algorithms | Risk management and anomaly detection |
How Machine Intelligence Transforms Credit Risk Evaluation in Crypto-Backed Lending
Blockchain-based lending platforms increasingly rely on machine learning algorithms to refine credit risk profiling. By analyzing on-chain behavior, wallet transaction history, and real-time token volatility, AI-driven systems build borrower models that go far beyond traditional credit scoring. This enables more accurate risk-based pricing and proactive exposure management for lenders operating in volatile crypto markets.
Predictive models trained on decentralized finance (DeFi) data also detect wallet behaviors associated with loan defaults, such as sudden token liquidations or abnormal staking patterns. These insights help lenders automate margin call triggers and optimize collateral thresholds, reducing exposure to market downturns and flash crashes.
Key AI Capabilities in Crypto Lending Risk Assessment
- Real-time sentiment analysis: Mining social media and news for market-moving narratives that affect asset risk.
- On-chain behavior profiling: Identifying borrower wallet patterns indicative of high-risk behaviors.
- Collateral token analytics: AI models assess token liquidity, volatility, and smart contract risks dynamically.
Note: Traditional credit checks are often useless in crypto lending; AI fills this gap with behavior-based risk analytics derived directly from blockchain data.
- Extract wallet-level financial signals using graph neural networks.
- Evaluate token-specific exposure via volatility clustering models.
- Trigger smart contract actions (e.g. auto-liquidation) based on risk thresholds.
AI Input | Risk Factor Assessed | Action Enabled |
---|---|---|
Wallet transfer patterns | Liquidity risk | Adjust collateral ratio |
Social sentiment shifts | Token trust degradation | Pause new loan issuance |
Historical lending behavior | Default probability | Set dynamic interest rates |
Leveraging AI Algorithms for Dynamic Crypto Expense Monitoring
In corporate finance operations involving cryptocurrencies, the challenge lies in accurately tracking decentralized expenditures across multiple wallets, exchanges, and blockchain layers. By applying predictive algorithms and data stream processing, organizations can monitor real-time asset flows, categorize transactions, and detect anomalies in spending behavior within seconds of execution.
Machine learning models trained on blockchain transaction patterns and historical budget allocations can forecast expenditure spikes and auto-adjust category thresholds, ensuring seamless reconciliation between crypto wallets and fiat accounting systems.
Core Components of AI-Powered Crypto Expense Management
- Automated classification: Neural networks identify transaction types (e.g., payroll, gas fees, asset swaps) using token metadata and address heuristics.
- Behavioral anomaly detection: Time-series models flag irregular wallet activity compared to baseline behavior.
- Multi-chain aggregation: Data pipelines collect expense logs from Ethereum, BNB Chain, and L2s into unified dashboards.
Note: Real-time analysis of on-chain expenses is critical for treasury departments operating across DeFi protocols and DAOs, where transaction volume can fluctuate rapidly.
- Deploy smart contracts that emit structured logs for ML consumption.
- Train models on synthetic datasets reflecting volatile crypto markets.
- Integrate outputs with ERP or internal ledger systems.
Component | ML Technique | Use Case |
---|---|---|
Transaction Clustering | K-means | Group wallet actions by purpose (e.g., vendor payments) |
Fraud Detection | Isolation Forest | Spot unauthorized fund movements |
Forecasting Gas Costs | LSTM Networks | Predict high-fee windows for batch operations |
AI Solutions for Identifying Illicit Activity in Blockchain-Based Corporate Payments
Large-scale financial operations increasingly involve blockchain transactions. With the anonymity and speed of crypto-based payments, corporate finance departments face significant challenges in identifying suspicious activities. AI-powered systems tailored to digital asset ecosystems now play a critical role in safeguarding institutional capital flows.
Modern machine learning engines utilize graph analytics, behavioral pattern recognition, and anomaly detection to trace the flow of digital assets through complex transaction networks. These tools integrate directly with crypto wallets, exchanges, and smart contracts, enabling real-time intervention and automated flagging of suspicious movement across ledgers.
Core Capabilities of AI Platforms in Blockchain Transaction Monitoring
AI-driven systems can identify hidden connections across thousands of pseudonymous addresses within seconds, reducing manual investigation time by over 90%.
- Transaction Chain Analysis: AI parses historical transaction flows to detect layering techniques used in money laundering.
- Address Risk Scoring: Algorithms assign risk scores to wallet addresses based on their interaction with known malicious entities.
- Real-Time Alerts: Systems trigger alerts when transactions match predefined risk signatures or deviate from expected patterns.
- Import blockchain data streams (Bitcoin, Ethereum, etc.) via API.
- Run clustering algorithms to identify associated wallets.
- Cross-reference with threat intelligence databases.
- Generate fraud risk profiles and alert compliance teams.
Tool | Key Feature | Use Case |
---|---|---|
Chainalysis KYT | Real-time risk monitoring | Crypto compliance in high-volume environments |
TRM Labs | Wallet clustering & behavioral analytics | Tracing cross-chain fraud schemes |
Elliptic Navigator | Forensics on mixer transactions | Uncovering obfuscated fund flows |
Streamlining Financial Statement Analysis with NLP in the Crypto Sector
Natural language processing has become a crucial tool for parsing complex financial documents in the crypto industry. With decentralized platforms generating large volumes of unstructured financial data–from token issuance reports to on-chain revenue summaries–manual review is no longer viable. NLP algorithms enable automated extraction of key financial insights from whitepapers, protocol audits, and earnings reports related to blockchain projects.
When applied to crypto-native financial disclosures, these models can identify subtle indicators of fiscal health, such as treasury depletion patterns or token burn schedules, which are often buried in textual narratives. This accelerates due diligence processes for institutional investors assessing DeFi protocols or NFT platforms for strategic entry points.
Key Use Cases of NLP for Crypto Financial Intelligence
- Parsing DAO treasury reports for liquidity trends
- Extracting staking reward distributions from validator logs
- Identifying financial red flags in smart contract audit disclosures
Note: NLP-driven classification models can flag rug-pull risk by detecting deceptive language patterns in whitepapers or anomalous tokenomics terminology.
Crypto Document Type | NLP Function | Insight Extracted |
---|---|---|
Tokenomics Section | Entity Recognition | Inflation control, vesting schedules |
Audit Report | Sentiment Analysis | Risk indicators, exploit likelihood |
DAO Proposals | Topic Modeling | Investment focus, cash flow allocations |
- Integrate NLP pipelines into crypto analytics dashboards.
- Train models on protocol-specific vocabularies.
- Continuously monitor on-chain text updates (e.g. governance votes).
Enhancing Budget Strategy with AI in the Cryptocurrency Sector
In the fast-paced world of cryptocurrency, the traditional methods of budget management are often inefficient and static, making them less suitable for the volatile nature of the market. By incorporating artificial intelligence into budget allocation processes, companies can create dynamic and adaptable financial strategies that are aligned with real-time market movements. AI tools can analyze large sets of data, including market trends, transaction volumes, and investor sentiment, to provide insights that drive more informed decision-making.
AI-powered systems are capable of continuously learning from the data they process, allowing businesses to adjust their spending, investments, and resource allocation instantly. This flexibility is crucial for navigating the often unpredictable cryptocurrency markets, where opportunities and risks emerge quickly. Below are some ways AI can optimize dynamic budget allocation in the cryptocurrency industry:
Key Benefits of AI for Budget Allocation in Crypto
- Real-Time Adjustments: AI can monitor market conditions 24/7 and make budget changes based on real-time data.
- Risk Management: AI tools predict potential market shifts and advise on how much budget should be allocated to safeguard assets.
- Data-Driven Insights: Through machine learning, AI can detect patterns that are invisible to human analysts, providing a more accurate financial forecast.
"By using AI-driven algorithms, companies can automatically allocate funds to the most profitable crypto assets while mitigating risks. This is especially useful for businesses that operate in fast-moving markets with high uncertainty."
AI Tools for Dynamic Budget Allocation
Tool | Functionality | Key Advantage |
---|---|---|
Cryptocurrency Portfolio Optimizer | AI-driven tool that dynamically adjusts portfolio allocations. | Real-time risk assessment and portfolio adjustment. |
Market Trend Analyzer | Analyzes trends in cryptocurrency prices and market sentiment. | Provides actionable insights for budget redistribution. |
Crypto Risk Management System | Utilizes AI to assess market volatility and allocate capital accordingly. | Helps mitigate the risk of significant financial loss. |
Improving Cash Flow Management Through Predictive Analytics in Cryptocurrency
In the volatile world of cryptocurrency, maintaining a stable cash flow is essential for financial planning and decision-making. Predictive analytics offers a powerful tool to help organizations forecast future cash inflows and outflows, minimizing risks associated with sudden market shifts. By analyzing historical data, algorithms can provide insights into potential trends and help businesses make informed decisions to optimize their cash flow strategies. This is especially important for crypto businesses, where fluctuations in asset prices can significantly impact liquidity.
Predictive models enable companies to anticipate short-term cash shortages or surpluses, ensuring they are prepared for market changes. In the cryptocurrency sector, this could mean being ready for a sudden spike in demand, a shift in token value, or a regulatory update. By implementing predictive analytics, crypto firms can better align their cash management processes with actual financial needs, reducing uncertainty and improving operational efficiency.
- Identifying patterns in transactional data
- Forecasting liquidity requirements based on market fluctuations
- Enhancing the accuracy of cash flow projections with real-time data
Key Insight: Predictive analytics allows for more accurate cash flow management by integrating blockchain data, transaction patterns, and external factors like market trends or regulatory changes.
- Utilizing blockchain data to forecast transaction volumes and timing.
- Implementing machine learning models for real-time cash flow predictions.
- Adjusting operational budgets and liquidity strategies based on predictive insights.
Example of Implementation:
Tool | Function | Impact |
---|---|---|
AI-driven liquidity forecasting | Predicts future token prices and liquidity needs | Improves decision-making by anticipating cash flow gaps |
Blockchain analytics | Tracks transaction trends and patterns | Enhances cash inflow predictions with real-time data |
AI Solutions for M&A Deal Evaluation and Scenario Planning in Cryptocurrency
In the fast-evolving cryptocurrency market, evaluating merger and acquisition (M&A) opportunities requires sophisticated tools capable of assessing complex data points. AI-driven solutions can significantly enhance decision-making by providing deep insights into the potential outcomes of a deal. Machine learning algorithms and predictive models can analyze vast amounts of blockchain data, market trends, and historical transaction records to evaluate the financial health of a target company or asset. This enables companies to assess risk, value, and strategic alignment more accurately before finalizing a deal.
Scenario planning powered by AI helps identify various future scenarios, from regulatory changes to market volatility, that could affect the outcome of an M&A transaction. By simulating different market conditions, AI tools help executives make more informed decisions on structuring deals, determining the right timing, and predicting post-deal integration challenges. These tools provide a higher level of certainty and confidence in a space where traditional financial modeling methods often fall short due to rapid market changes.
- Analyzing target company blockchain activity and transaction trends
- Identifying synergies and potential risks based on historical market performance
- Simulating multiple market scenarios to assess deal outcomes
Insight: AI can process large volumes of cryptocurrency-related data, such as token price fluctuations, to identify potential M&A targets with favorable risk profiles and strategic fit.
- Using AI to evaluate the financial health and market position of a target firm.
- Implementing scenario modeling to understand potential outcomes under different market conditions.
- Optimizing deal structures by simulating post-transaction performance and integration challenges.
Example of Implementation:
Tool | Function | Impact |
---|---|---|
AI-powered deal evaluation platform | Analyzes blockchain data and market trends for M&A insights | Enhances the accuracy of risk and value assessments |
Scenario simulation software | Models different market conditions and their impact on deals | Helps determine optimal deal timing and structure |